In many ways, treasure hunters are like data teams, who also attempt to discover hidden insights in a vast area of enterprise data.
Treasure hunters have their bag of tools, everything from GPS and navigation tracking tools to shovels and brushes. Similarly, enterprise data teams rely on data observability to improve data discovery so they can eliminate the need to manually locate files, update catalogs, and maintain metadata logs - all which leads, ultimately, to better data value. With data observability, data engineers uncover insights that lead to better return on their data investments and increased data value, whether through cost optimization, performance management, and/or improved data reliability.
Data teams that are using data observability recognize that uncovering value happens in continuous fashion. The Greek philosopher, Heraclitus, said that one never steps in the same river twice. It’s an astute paean to the notion of change and evolution. Similarly, as data environments are in constant change, data teams are looking at and evaluating data in a continuously evolving state. There are a lot of tools that help them see what’s happening, but only data observability helps them accurately pinpoint where changes need to be made and advantages can be capitalized upon in order to uncover data value.
Data Silos Can Hinder Cross-Functional Data Insights
Discovering the right data is a big problem because information, by default, gets siloed in different databases within your enterprise. As a result, you can spend over five hours every week waiting to access existing information or recreating it.
Different teams within a large enterprise also often create different data sets. Marketing may create campaigns based on user web interaction data. Product may create a roadmap of new improvements and features based on user behavior data. And customer support may create voice scripts based on support request data.
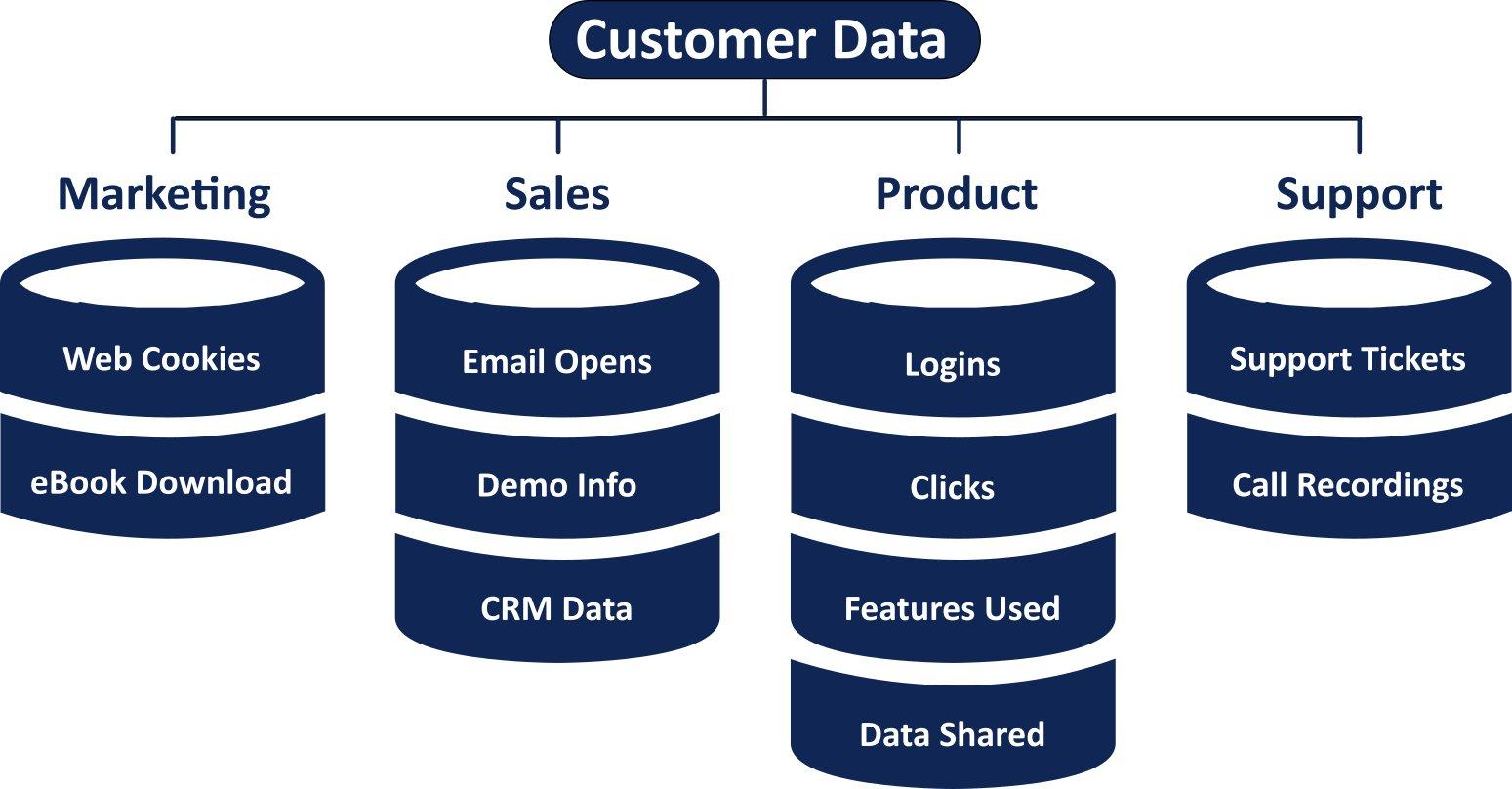
But seldom do these data points get shared between departments and teams. And even more rarely do enterprises use these cross-functional data across different teams to improve business outcomes or customer experience. As a result, they can lose out on opportunities to highlight product features or recommend product upsells to the right users.
Manual Code, Catalogs, and Metadata Work — But Aren’t Scalable
Data sources require that engineers write custom scripts that can help you find the data you need. But these manual processes aren’t scalable — nor can they keep pace with the increasing volume, variety, and velocity of incoming data. As a result, a big chunk of your data will remain hidden from people who can act on it.
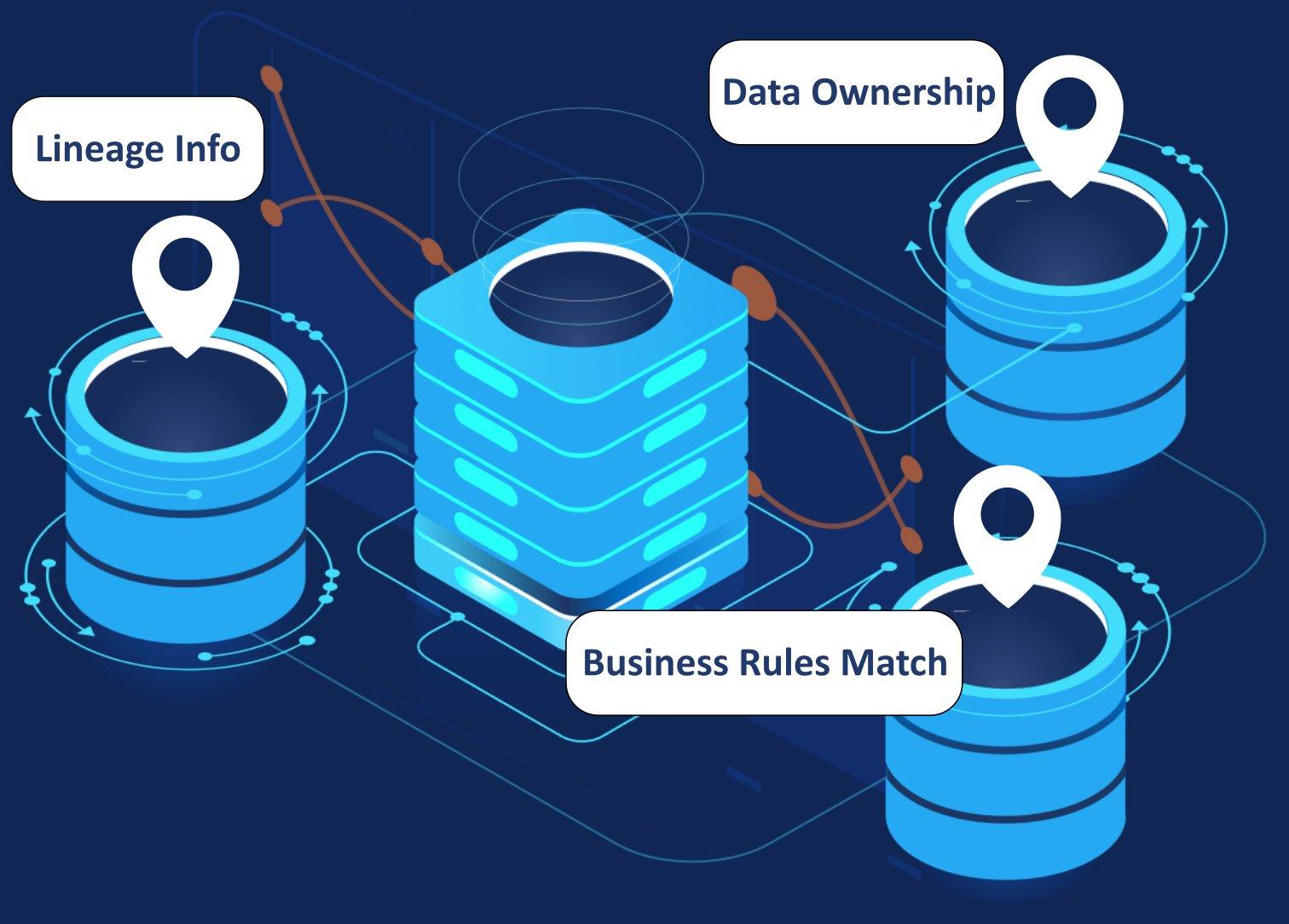
Data catalogs, metadata logs, and custom scripts can help you go beyond organizational data silos to discover the right data. But they take up the time of data engineers, who already spend between 25% - 40% of their time collecting and gathering data. Then, an inordinate amount of time is spent cleaning all of that data.
Overworked data engineers simply don’t have the time to manually create scripts, catalogs, and metadata logs across all data sources. So you need new ways to improve data discovery at scale.
Data Observability Improves Data Discovery at the Required Scale
In addition to helping you understand your data life cycle and data environment, data observability can also identify data quality and reliability problems. It can automatically check for schema and data drift. And it can reconcile data in motion.
A data observability solution like the Acceldata Data Observability Platform, automatically scans your data environment and offers powerful profiling capabilities to monitor enterprise data across all your data lakes, warehouses, and repositories.

Acceldata shows how your data gets transformed along the entire data life cycle from ingestion all the way up to consumption. It also offers a unified view of your data pipeline across multiple technologies. You can see here how data teams can identify the different data sources and how they’re operating:
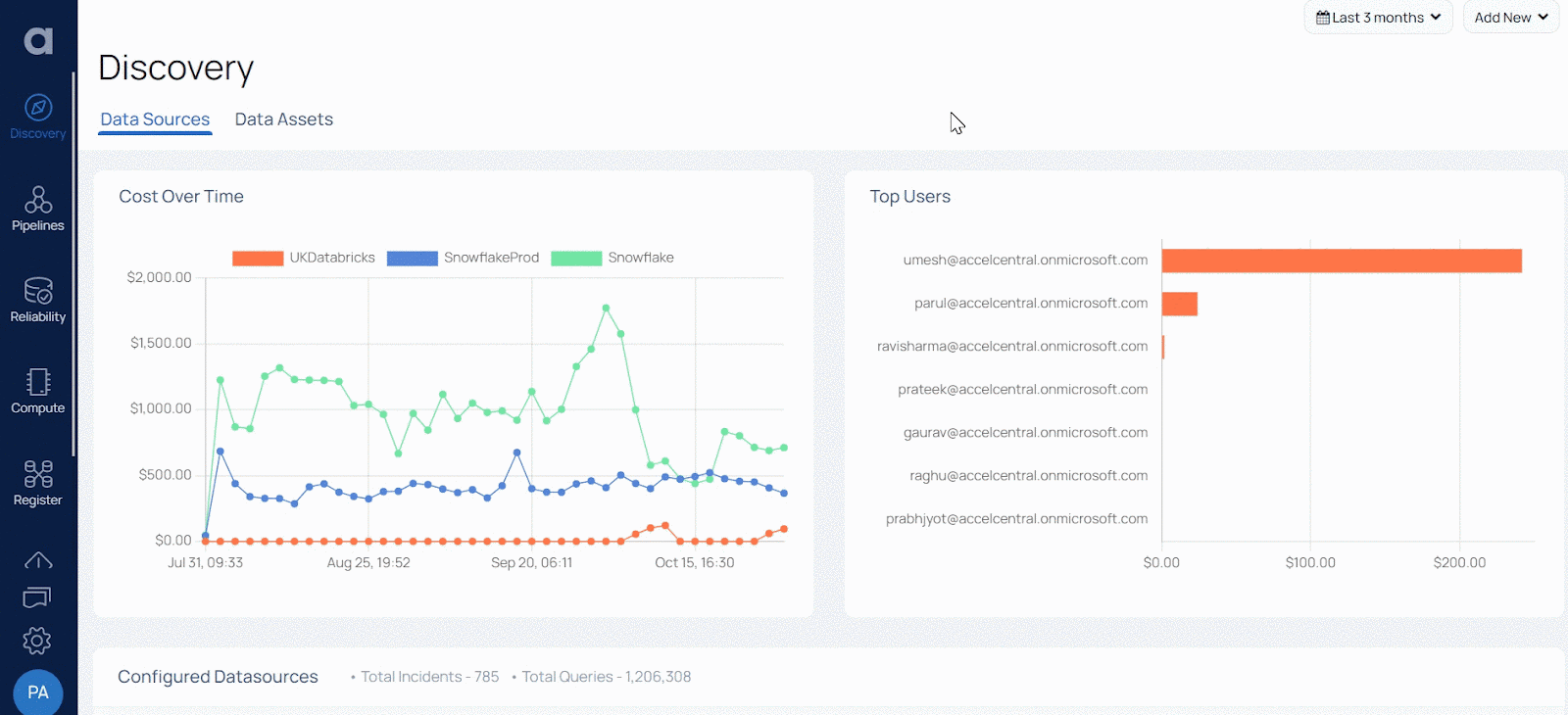
Acceldata also helps you classify, catalog, and manage business rules for both data at rest as well as data in motion. It automates data discovery, exploration, and validation across all your sources — helping you identify and validate data quickly at scale.

Acceldata can help reconcile data in motion or during cloud migrations to ensure that data arrives as expected. It can automatically detect schema or data drift. Its comprehensive rules engine automatically checks for missing or incorrect data. It also offers self-service catalogs and metadata profiles to help you discover data rapidly across all your data sources.
Increase Data Value with Data Observability
Advancements in data observability now allow you to eliminate time-consuming and plodding manual processes of finding the data you need. Acceldata’s advanced profiling capabilities can automatically update data catalogs and metadata profiles across all your data sources, making them rapidly discoverable.
Get a free personalized demo of Acceldata and see how data observability can help your organization.