In today's dynamic landscape of data management and analytics, guaranteeing the integrity, quality, and dependability of data is of utmost importance. In this multi-cloud, multi-technology data-driven world we are in now, as our visionary co-founder and CEO, Rohit Choudhary mentioned in his NYSE interview: data nowadays traverses through a very complex journey from ingestion and all the way to consumption - through multiple layers of varied technologies and transformations. Ensuring quality and reliability of data in this end-to-end journey is key.
Time to get your ducks in a row! Time for enterprises to get your data right!
Acceldata stands out by offering advanced data observability functionalities such as anomaly detection - strategically integrated at various places throughout the Acceldata platform - empowering enterprises to continually and proactively ensure data quality across the end-to-end data landscape.
Let’s explore Anomaly Detection in Acceldata's Data Observability Cloud (ADOC) in this blog.
Understanding Anomaly Detection
Anomaly detection is the process of identifying patterns or behaviors in data that deviate significantly from the norm. This involves the systematic identification and resolution of anomalies - irregularities or outliers - within the datasets.
An anomaly refers to any deviation or irregularity in data that does not conform to expected patterns or behaviors. These anomalies can arise due to various reasons such as errors in data collection, data entry mistakes, technical glitches, or genuine outliers in the data.
Detecting and handling anomalies is crucial for ensuring data quality and reliability. These anomalies, if left unaddressed, can undermine the accuracy of analyses and decision-making processes based on this data.
Anomaly Detection in Acceldata's Data Observability Cloud (ADOC)
In ADOC, the anomaly detection capability is built on highly advanced algorithms and machine learning models meticulously crafted by experienced data engineers, with several years of data experience, and refined over time through invaluable customer feedback garnered from numerous enterprise clients spanning various industries.
By continuously monitoring various data metrics and dimensions, ADOC has the capability to swiftly pinpoint anomalies in real-time. This proactive approach enables our customers to address potential issues promptly, safeguarding the integrity and security of their data landscapes.
Handle Modern Data Complexity with Advanced Data Observability Solution
With increasingly varied data sources and ever-evolving schemas, most data observability platforms fail to appropriately detect anomalies and trends that ultimately cost the company time and money.
Data behaves dynamically, so why are so many of your insights seemingly static? Why settle for subpar data observability solutions? More likely than not, your limited analytics stem from your platform’s built-in limits around data profiling and historical context.
Acceldata provides the following advanced anomaly detection capabilities in its data observability platform.
ML-based Anomaly Detection Capabilities
ML-based anomaly detection capabilities (for example: anomalies related to Data Freshness and Data Profiling) help identify unexpected changes in data which may not be covered by an explicit business rule validation in the platform.
For example, you can identify the unexpected items or events in a dataset with the historical parameters. Anomaly detection is the process of checking the values in a dataset for irregularities by using historical metrics.
This AI/ML-based anomaly detection feature is a proactive approach to maintaining data quality and integrity, enabling organizations to swiftly respond to potential problems before they escalate.
Application of Anomaly Detection Across the ADOC Platform
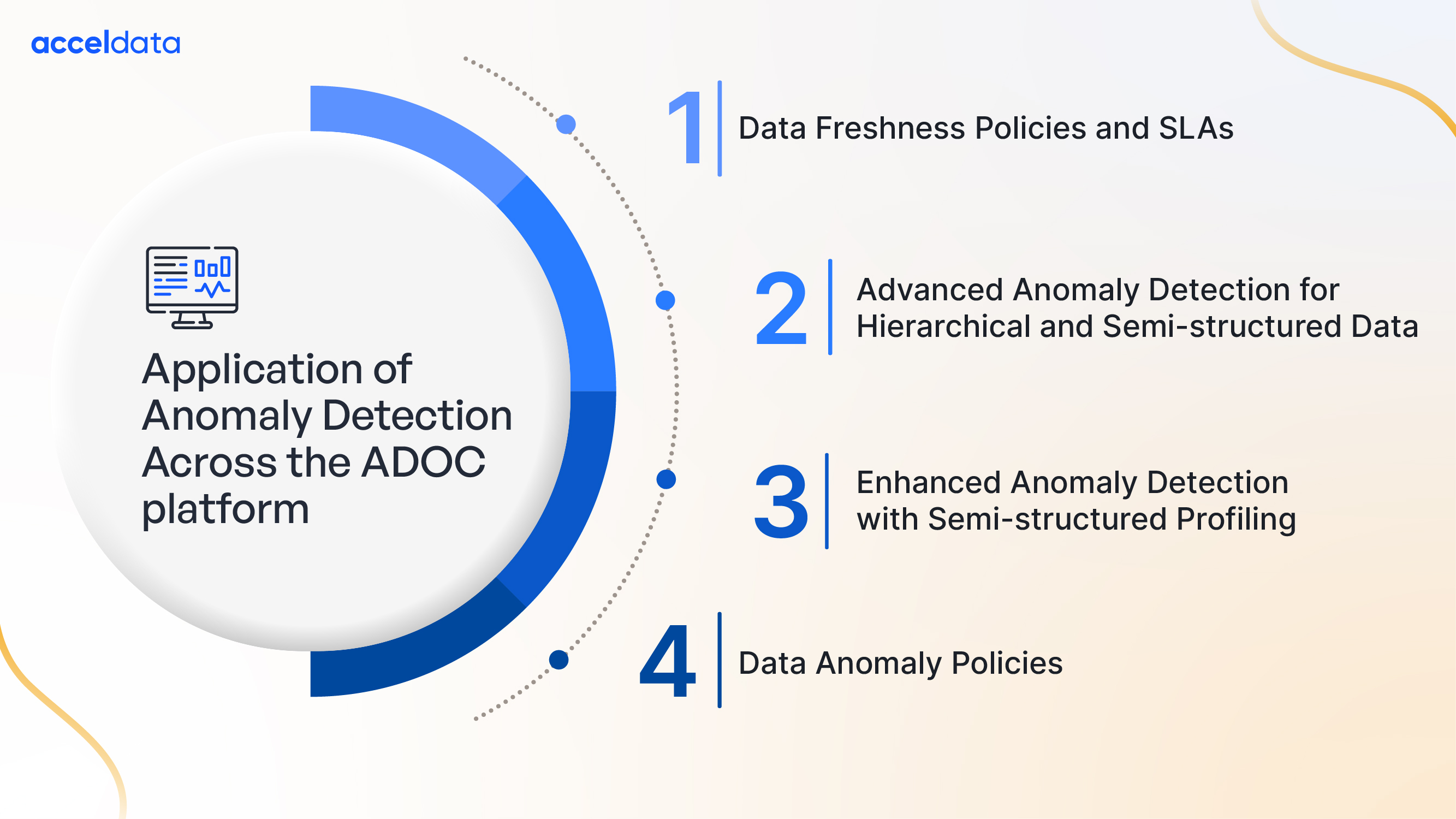
Enhancing the reliability of data involves studying and alerting on anomalies in data freshness, data profiling, and data quality changes.
ADOC applies advanced anomaly detection in several use cases throughout the data landscape, such as:
- Data Freshness policies and SLAs
- Data Anomaly policies
- Enhanced Anomaly Detection with Semi-structured Profiling
- Advanced Anomaly Detection for Hierarchical and Semi-structured Data
Let’s explore each of these scenarios that are significantly enhanced by advanced anomaly detection in ADOC in the following sections:
Anomaly Detection Applied in Data Freshness Policies and SLAs
The data freshness policy in ADOC plays a crucial role in ensuring that your data remains current and up to date. Freshness is of utmost importance, especially for real-time analytics and other time-sensitive applications.
By implementing the data freshness policy, ADOC continuously monitors the freshness of your data and alerts you to any inconsistencies or delays in data delivery.
This helps you maintain the integrity and reliability of your data, ensuring that it is always up to date and suitable for critical operations.
Real-time analytics heavily depend on the timeliness of data to provide accurate insights and enable prompt actions. Similarly, other time-sensitive applications require up-to-date data to function effectively.
Additionally, anomaly detection for data freshness policies provides a mechanism for ADOC to automatically learn, monitor, and alert on unexpected occurrences related to the delivery of data. These policies proactively ensure that your data is always current and up-to-date, which is crucial for real-time analytics and time-sensitive applications.
In addition to the anomaly detection framework, users can also specify organizational SLAs around these metrics. SLAs provide a non-model based approach to monitoring and alerting, aligned with your business goals. You can define thresholds or rules for data freshness and other metrics, and ADOC will monitor and alert you when these thresholds are breached.
By setting up Data Freshness policies and defining SLAs, you can ensure that your data meets the required freshness criteria and is delivered within the specified timeframes. This helps you maintain the reliability of your data and meet your business objectives.
Data Anomaly Policies
Acceldata's AI/ML-based anomaly detection is a powerful feature that helps in identifying unexpected changes in your data. This is achieved through the implementation of Data Anomaly Policies.
Data Anomaly Policies automatically learn and then monitor and alert on unexpected changes to the underlying data set.
As data is continuously profiled, users can be notified when key profiling metrics change from the norm. A variety of metrics are available for monitoring based on the underlying data type of the profiled data.
Anomaly detection enables the Acceldata platform to monitor data without any input from users (ie: rules, code) to identify issues automatically as data or pipelines changes unexpectedly.
Data Anomaly policies are particularly valuable in scenarios where explicit business rule validations may not cover all possible data anomalies. These policies provide an additional layer of monitoring and validation, helping you maintain data integrity and compliance with regulatory requirements. By automatically learning and alerting on unexpected changes in the underlying data set, Data Anomaly policies contribute to your data governance efforts and support effective decision-making.
Enhancing Anomaly Detection with Semi-Structured Profiling
The latest version of ADOC has expanded its capabilities to include the profiling of semi-structured data. This means that ADOC can now detect anomalies and provide comprehensive data quality and integrity insights for semi-structured data types.
Previously, ADOC's profiling capabilities were limited to simple data types. However, with this enhancement, ADOC can now analyze and identify anomalies in semi-structured data profile metrics, giving you a more comprehensive view of the quality and integrity of your data.
This is particularly valuable as semi-structured data, such as JSON or XML, is becoming increasingly prevalent in modern data ecosystems. By being able to profile and analyze this type of data, ADOC empowers you to maintain and improve the overall quality of your data assets.
Advanced Anomaly Detection for Hierarchical Data
The Acceldata ADOC platform has been deliberately designed to be sophisticated enough to not just identify anomalies in hierarchical data, but also trace potential impacts of anomalies or outliers up or down the hierarchical structure, thus assuring data quality and improving the debugging process.
Through automatic struct profiling and flattening depth configuration, Acceldata ensures the accurate representation of hierarchical data stored in JSON-based assets, avoiding the loss of nested information or data integrity issues commonly associated with flattening arrays. By retaining the inherent structure of arrays within Snowflake, Acceldata enables organizations to preserve the richness and depth of insights, thus enhancing data observability and analysis.
Acceldata thus offers robust support for hierarchical and semi-structured data formats, particularly in conjunction with data stores - such as Snowflake. Unlike many observability platforms, Acceldata recognizes the complexities of sophisticated data stores (such as Snowflake) and is designed to handle the intricacies of auto clustering and the semi-structured data it houses.
Acceldata stands out among observability solutions for its adeptness in managing structured hierarchies across diverse formats like JSON, parquet, and relational databases, facilitating tasks such as relationship mapping and entity dependency comprehension.
By tracing potential impacts of anomalies or outliers throughout the hierarchy, Acceldata not only assures data quality but also streamlines the debugging and issue remediation process, ultimately improving overall data observability and MTTR.
Advantages of Anomaly Detection in Data Observability
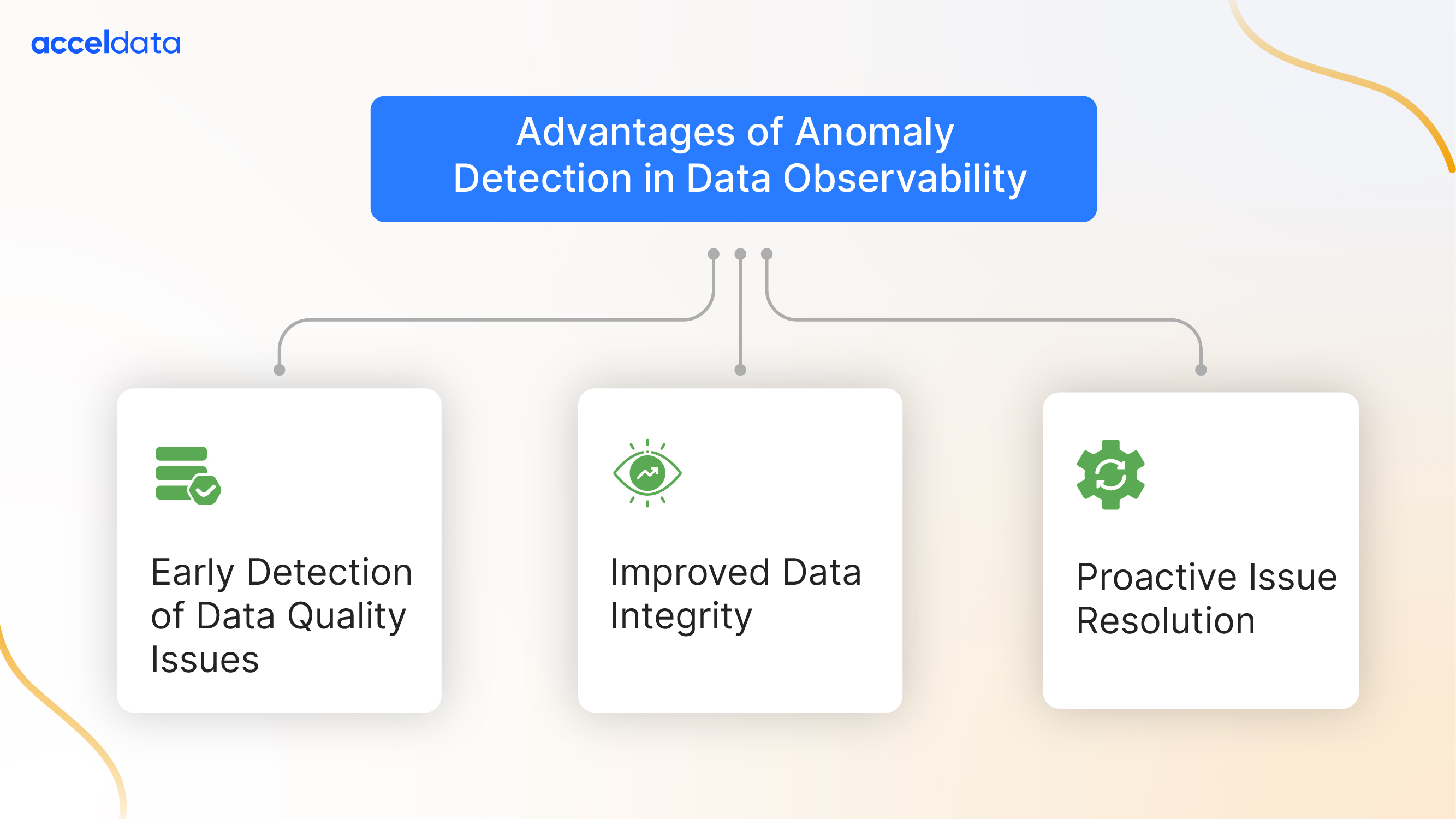
- Early Detection of Data Quality Issues: Timely identification of anomalies allows organizations to promptly address data quality issues, ensuring accurate decision-making.
- Improved Data Integrity: Continuous monitoring and analysis of data ensure high standards of data integrity, instilling confidence in analytical processes.
- Proactive Issue Resolution: Early anomaly detection enables organizations to proactively resolve potential problems, minimizing disruptions and downtime.
Summary
Anomaly detection thus plays a crucial role in data observability by enabling timely identification of irregularities and deviations within datasets. By proactively flagging potential issues, anomaly detection enhances data quality, fosters accurate decision-making, and facilitates proactive problem resolution, ultimately ensuring the reliability and trustworthiness of organizational data.
Anomaly Detection in Acceldata ADOC empowers organizations to maintain data quality, integrity, and reliability - at scale, and on a continual and preventive basis. By leveraging advanced algorithms and machine learning models, ADOC enables real-time anomaly detection, ensuring prompt issue resolution and optimized decision-making processes.
Enterprises gain deeper insights into their data - no matter how complex the data may be, or no matter where it is on its journey from ingestion to consumption. These proactive measures maintain data integrity and compliance, by design, rather than a reactionary measure.
Acceldata's commitment to continuous innovation ensures that ADOC remains at the forefront of data observability solutions, driving success for data-driven enterprises.