In today's digital age, data has become a critical asset that guides the direction of businesses of all sizes. Organizations rely on data to gain insights into customer behavior, improve operational efficiency, and make informed business decisions. However, the value of data is only as good as its quality and reliability.
Poor data quality and reliability can lead to incorrect or incomplete analysis, which can have serious consequences for the business. Hence, mastering data quality management has become imperative for businesses aiming to harness the full potential of their data assets.
What is Data Quality Management?
Data Quality Management (DQM) encompasses a specific set of practices designed to enhance the quality of data utilized by businesses. At its core, data quality refers to several critical dimensions: accuracy, completeness, consistency, timeliness, relevance, and validity of data. Each of these dimensions plays a crucial role in ensuring that data can be trusted and utilized effectively for decision-making purposes.
The Importance and Impact of Data Quality Management
Research has repeatedly demonstrated that poor data quality management can have significant financial repercussions for businesses. In 2016, IBM released a report estimating that the cost of bad data for U.S. businesses was around $3.1 trillion dollars annually. As businesses continue to manage increasing volumes of data, that number is only likely to rise. Data Quality Management is the solution to this problem, providing a framework to ensure data integrity and reliability, which is essential for making informed business decisions and minimizing financial losses associated with poor data quality.
Key Dimensions of Data Quality Management
Accuracy:
It measures how closely data reflects the real-world objects or events it represents. Inaccurate data can lead to faulty analyses and misguided decisions.
Completeness:
In data quality, completeness refers to whether all required data is present. Incomplete data sets may skew analyses and hinder comprehensive insights.
Consistency:
It helps to ensures that data is uniform and coherent across different sources and systems. Inconsistent data can result in discrepancies and confusion.
Timeliness:
This aspect of data quality indicates whether data is up-to-date and relevant for the task at hand. Outdated data may lead to obsolete insights and missed opportunities.
Relevance:
It assess the appropriateness of data for a given purpose or context. Irrelevant data can distract from meaningful analysis and decision-making.
Validity:
This determines whether data conforms to predefined rules and constraints. Invalid data can compromise the integrity of analyses and undermine trust in the data.
Challenges in Data Quality Management
.webp)
Despite its importance, organizations faces various data quality issues. Maintaining high data quality poses several challenges like:
Data Duplication:
Duplicate records can proliferate across databases, leading to inconsistencies and inefficiencies.
Inconsistent Data Entry:
Human error during data entry processes can result in inconsistencies and inaccuracies.
Lack of Data Standardization:
The absence of standardized formats and conventions makes it difficult to integrate and analyze data effectively.
Data Silos:
Data stored in isolated systems or departments inhibits collaboration and hampers data quality efforts.
Poor Data Governance:
Inadequate governance structures and processes weaken accountability and oversight of data quality initiatives.
Best Practices for Data Quality Management
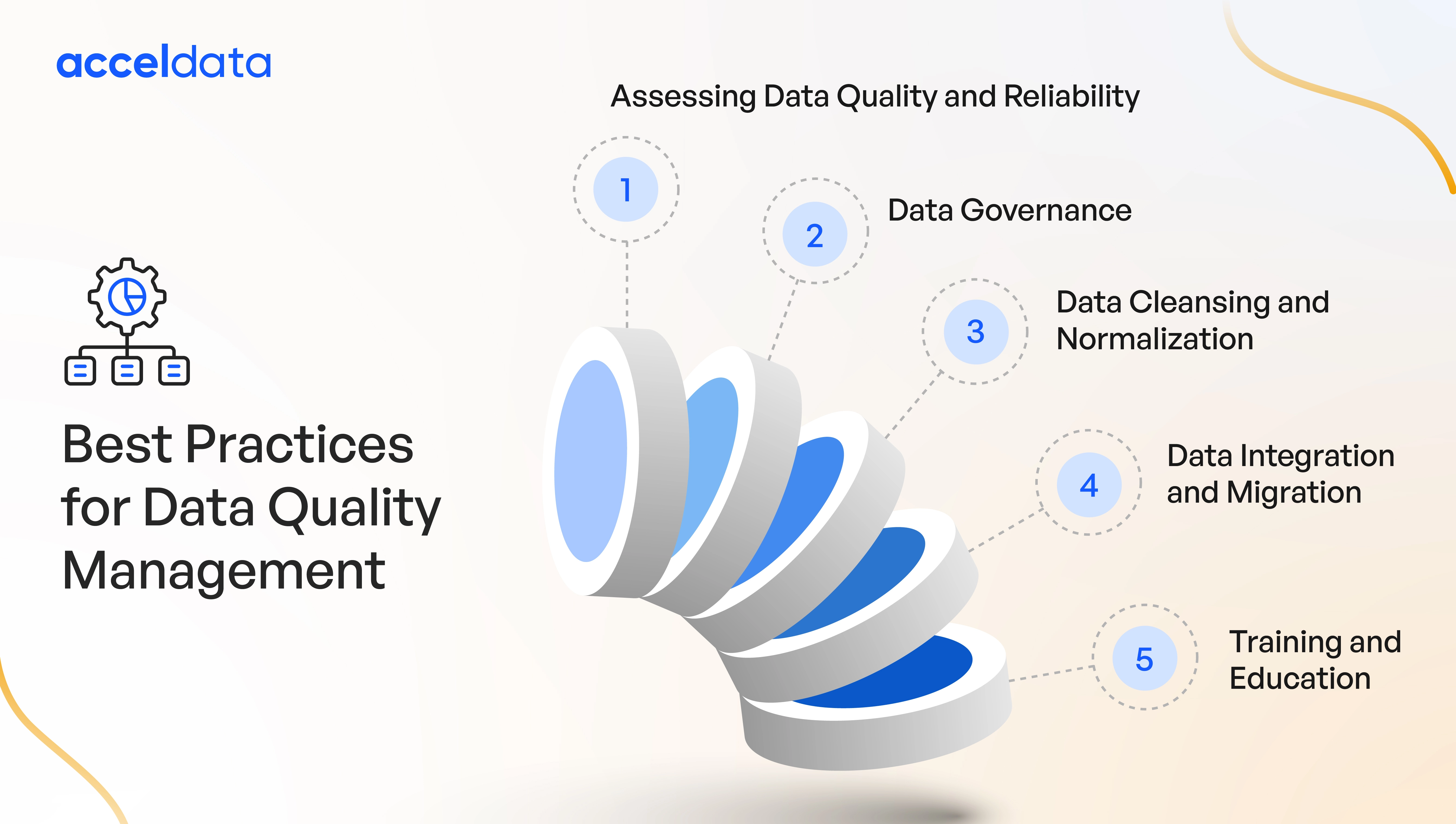
The increase in the demand for data in the contemporary digital age has led to a remarkable challenge - a data crisis. It results from poor-quality data which makes it unusual or unreliable for businesses. Data Quality Management prevents these crises from occurring by providing a quality-focused foundation for an enterprise’s data.
Data Quality Management has become an essential process that helps businesses to make sense out of data. It helps to ensure that the data present in systems is appropriate to serve the purpose. Here are the best practices for data quality management.
Assessing Data Quality and Reliability
The first step towards improving data quality and reliability is the assessment of the current state of your data. You can start by identifying the sources of the data, which includes both internal and external sources, such as customer data, sales data, and third-party data. Once you identify your data sources, you can evaluate the data for completeness, accuracy, consistency, timeliness, and validity. This will help you identify areas for improvement and develop a plan for data cleansing and normalization.
Data Governance
This practice in data quality management plays a critical role in maintaining data quality and data reliability. It involves establishing a data governance program to ensure proper management, security, and quality of data. This includes developing policies and procedures for data management, assigning roles and responsibilities, and ensuring compliance with relevant regulations and standards.
Data Cleansing and Normalization
One of the most effective ways to improve data quality and reliability is through data cleansing and normalization. This involves identifying and correcting errors and redundancies in data, standardizing and normalizing data formats and structures, and removing duplicates. Data cleansing and normalization can improve data accuracy, consistency, and completeness, which are essential for reliable data analysis.
Data Integration and Migration
Integrating data from multiple sources and migrating it from legacy systems can be a complex process. However, it's essential to ensure data quality during integration and migration to avoid data inconsistencies and errors. This involves selecting appropriate tools and technologies for data integration and migration, testing and validating data before and after migration, and ensuring data security during the process.
Training and Education
Finally, training and education play a crucial role in maintaining data quality and reliability. It's important to train employees on data management and governance best practices and provide ongoing education to keep them up-to-date with new technologies and regulations. Sharing best practices for maintaining data quality and reliability can also help organizations avoid common pitfalls and ensure maximum value from their data assets.
Data Quality Management Framework
An effective data quality management framework is comprehensive, covering all six main pillars of data quality and the entire data cycle from the source to analysis by end-users. This includes establishing policies for data handling. Over 70% of employees currently have access to data they shouldn't, an issue solvable only with solid data observability.
For example, Acceldata automates much of your data quality management with an AI system that improves dynamic data handling and detects invisible errors like schema and data drift. Referring to a data quality framework implementation guide can help you learn about the essential checks for effective data quality management.
Data Quality Management Examples
Practical Perspectives on Data Quality Management
We’ve discussed much of the theory surrounding good data quality management. However, from a practical perspective, what does this process actually look like? What are some data quality management examples?
Data Profiling
One great example of data quality management is data profiling. Data profiling is the process of monitoring and cleaning up data to enable organizations to make better, insights-driven decisions. Traditionally, data profiling is a complex, time-consuming task requiring hours of manual labor. However, with Acceldata, you can introduce automation into the process, freeing up your teams to focus on analysis and decision-making instead of cleaning and organizing.
Additional Examples of Data Quality Management
Other data quality management examples include smart data handling policies and data validation tasks. These practices ensure data is processed and used correctly, minimizing errors and enhancing overall data quality.
Advanced Features of Acceldata
Machine Learning and Dynamic Recommendations
Acceldata enables you to get the context you need with machine-learning-based classification, clustering, and association. Plus, it offers a dynamic recommendations feature that can help you rapidly improve data quality, accuracy, coverage, and efficiency.
Building a Data Quality Management Framework
To implement effective data quality management, a structured framework is essential. This framework ensures that all aspects of data quality are addressed comprehensively.
Definition and Leadership Buy-In
Good data quality starts with a data quality management definition. This definition should have buy-in from your company’s top leadership.
Roles, Responsibilities, and Tools
The framework you build and implement needs to clearly define the data quality management roles and responsibilities as well. Furthermore, your plan should also describe the kinds of data quality tools that are going to be used.
Future Trends in Data Quality Management
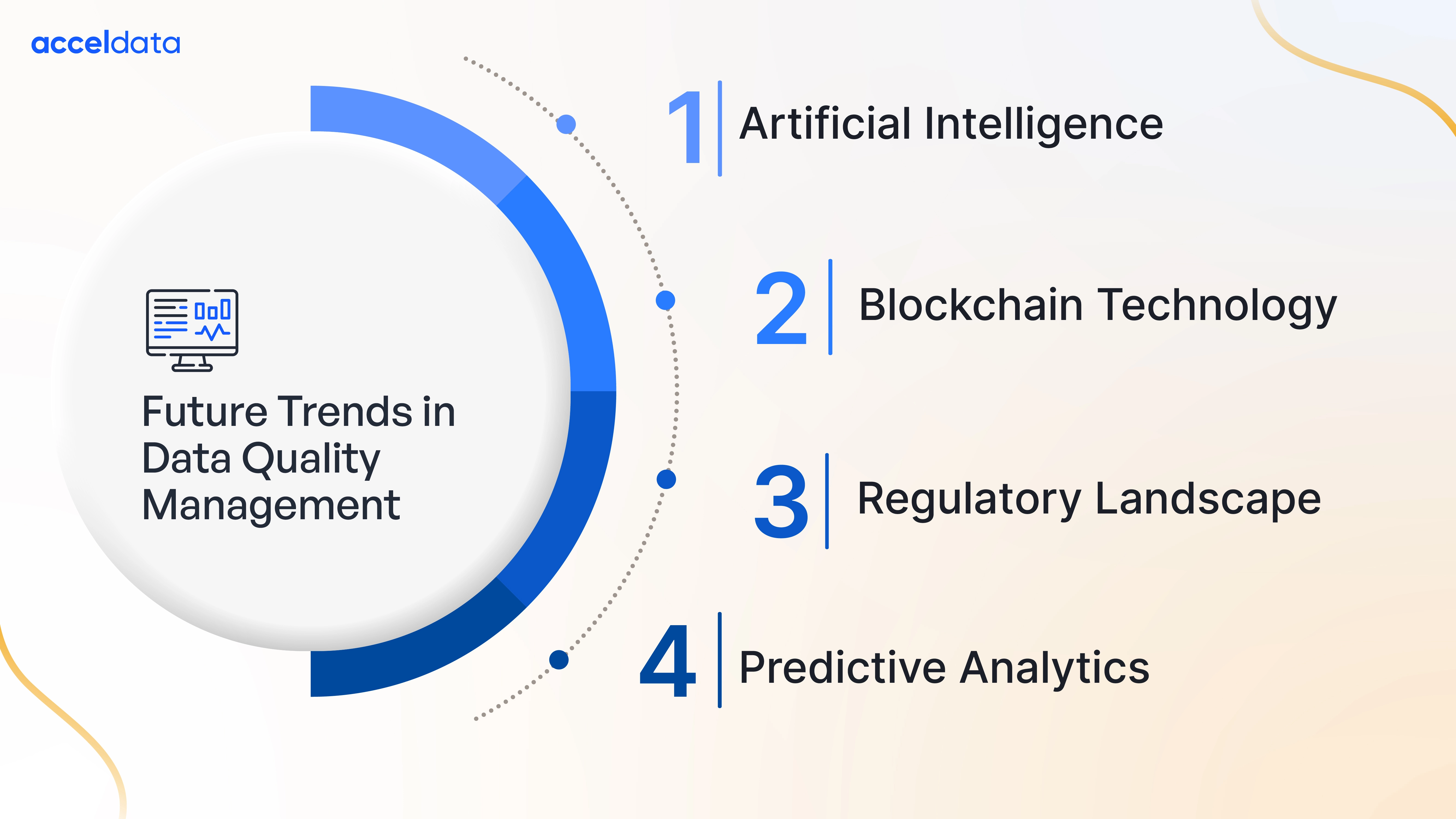
As technology continues to evolve, several trends are shaping the future of data quality management:
Artificial Intelligence and Machine Learning:
AI and ML algorithms are increasingly being used to automate data quality processes, detect anomalies, and improve data cleansing techniques.
Blockchain Technology:
Blockchain offers enhanced data integrity and security, reducing the risk of data tampering and ensuring trust in digital transactions.
Regulatory Landscape:
Evolving regulatory requirements, such as GDPR and CCPA, are placing greater emphasis on data governance and compliance, driving organizations to prioritize data quality management.
Predictive Analytics:
Predictive analytics enables organizations to anticipate and prevent data quality issues before they occur, enabling proactive management of data quality.
Data Quality Management: Leveraging Acceldata Data Observability
In today’s highly competitive business environment, knowing how to get the most out of your data is crucial. However, making a decision based on poor-quality data can lead to massive problems. The reality is that big data is called that for a reason. Most enterprises have to manage thousands of different sources and hundreds of thousands of data points that all need to be correctly organized and monitored to ensure their reliability and quality. You cannot maintain the quality of something you cannot see. That’s why data observability is so crucial to data quality management.

Importance of Data Observability
Ensuring data quality management with data observability involves implementing robust processes and technologies to continuously monitor, analyze, and optimize the quality of data across its lifecycle. By leveraging Acceldata data observability organizations can gain real-time insights into the health, performance, and reliability of their data pipelines, systems, and workflows. It provides visibility into data lineage, dependencies, and transformations, allowing teams to identify anomalies, errors, and deviations from expected behavior.
Benefits of Proactive Data Management
Moreover, data observability enables proactive detection and resolution of data quality issues, minimizing the impact on downstream processes and decision-making. By establishing a culture of data transparency, accountability, and collaboration, organizations can harness the power of data observability to ensure high-quality data that drives accurate insights and informed decisions.
Final Words
Mastering data quality management is essential for organizations seeking to unlock the full potential of their data assets. By understanding the dimensions of data quality, addressing common challenges, adopting best practices, and embracing emerging trends, businesses can ensure data integrity, reliability, and relevance in an increasingly data-driven world. Ultimately, prioritizing data quality management is not just a necessity but a strategic imperative for success in the digital age.