Data isn't just information. It's an asset you need to protect and maintain. Without systems to monitor the quality of your data, it can rapidly change from an asset to a liability. But the volume and speed of data is always growing, which means the margin of error is shrinking. What can you do to be sure that your analysis is steering you in the right direction?
Let's look at five of the most common data quality issues, and how you can prevent, detect, and repair them.
What Is Data Quality?
Can you trust your data? How well do your datasets suit your needs? Data quality quantifies the answers to these questions. Your data needs to be:
- Accurate: It must correctly and precisely represent the values it claims to.
- Complete: All the required data must be present.
- Valid: The values represented in the data are consistently defined and represented.
- Timely: The information is up to date.
- Relevant: The data meets its intended purpose.
These factors impact the reliability of the information your organization uses to make decisions. Unless you set standards and monitor the quality of your data, your ability to rely on it is, at best, suspect. Data quality is critical because reliable information is crucial to business success. Without quality data, quality decisions are impossible.
Five Common Data Quality Issues
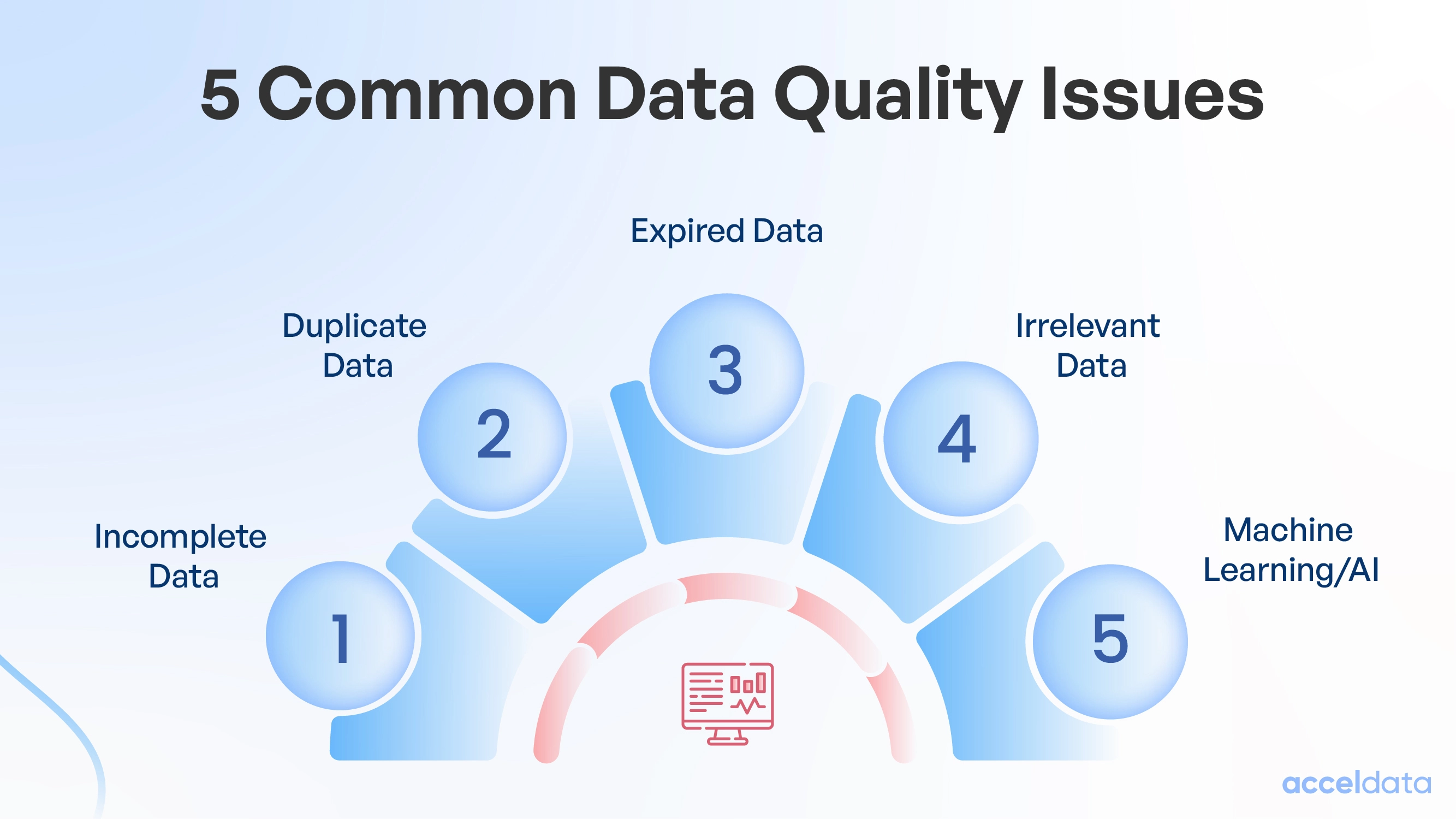
Let's delve into how you can use the criteria above to monitor your data and identify specific data quality issues.
1. Incomplete Data
Data is incomplete when it lacks essential records, attributes, or fields. These omissions lead to inaccurate analysis, and ultimately, incorrect decisions. So, you need to not only avoid incomplete data but also be aware of when it inevitably occurs.
Incomplete data is often caused by:
- System failures: When a collection process fails, it can cause data loss.
- Data entry errors: Sometimes data is entered incorrectly or omitted. This is especially common with manually entered information.
2. Duplicate Data
Data is duplicated when the same piece of information is recorded more than once. If not detected, duplicate data skews analysis, causing errors like overestimation. This problem can occur when you initially acquire data or when you retrieve it from your internal storage.
Duplicate data results from:
- Data entry errors such as recording the same record twice.
- Collecting data from more than one location or provider and not properly filtering it.
- Metadata issues that lead to cataloging and storage mistakes.
- Inefficiencies in data architecture that result in data being stored incorrectly or in more than one location.

3. Expired Data
Expired data is out of date; it no longer represents the current state of the real-world situation in the data models. How quickly data expires or goes stale, depends on the domain. Financial market data can be updated more than once a second. Client address and contact information may only be updated a few times a year.
When it goes undetected, expired data is especially problematic because at some point it was accurate, so it may pass naive quality checks. This leads to an analysis that is, similar to the input and is no longer accurate. Data expires or goes stale when it isn't updated on time. This happens because of data acquisition errors, poor data management, or entry errors.
4. Irrelevant Data
Data that doesn't contribute to your analysis is irrelevant. Unneeded data is collected when you don't target your gathering efforts well or don't update them to meet new requirements.
Collecting extra information because it may be useful later seems proactive and strategic. However, storing irrelevant information is rarely a good idea. In addition to placing extra stress on collection and storage systems and increasing costs, it increases your security risks, too.
Irrelevant data proliferates when collection is poorly targeted and when data stores are not pruned based on data aging and changing requirements.
5. Inaccurate Data
Inaccurate data fails to properly represent the underlying information. Like duplicate, expired, and incomplete data, inaccurate data leads to incorrect analysis.
Many factors cause inaccuracies, including human errors, incorrect inputs, and data decay, which is a type of expired data.
Avoiding Common Data Quality Issues
Each of these issues has a detrimental impact on your data analysis, and ultimately, your ability to make accurate decisions. So how do you avoid them? How can you be sure you're using high-quality data that stays that way?
.webp)
Data Governance
Ensuring data quality starts and ends with governance. Without a comprehensive program to manage the availability, usability, integrity, and security of your data, the best tools on the market will fail. Data governance collects your data practices and processes under a single umbrella.
Data Quality Framework
You can't catch quality issues without a structured set of guidelines and rules that define what accurate, reliable, and useful data is. A data quality framework includes these guidelines, as well as the processes, methods, and technologies you use to enforce them.
Your framework should include:
- Data Quality Standards: Clear definitions of the completeness, consistency, timeliness, and relevance that your data must meet.
- Data Quality Measurement: How you'll assess and track the quality of your data, often through specific metrics or key performance indicators (KPIs).
- Data Quality Assurance: The ongoing activities you'll maintain to ensure your data continues to meet quality standards over time. This includes audits, validation, and other monitoring efforts.
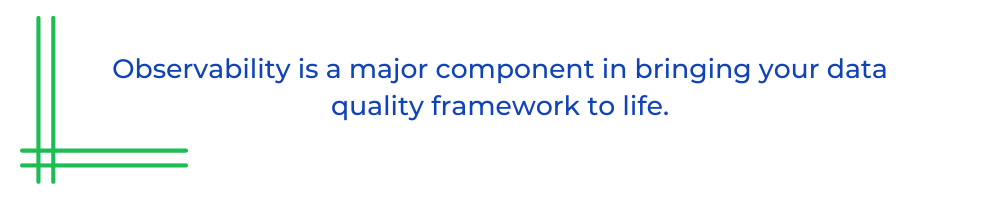
Data Observability
Observability is a major component in bringing your data quality framework to life. It gives you the ability to see the state and quality of your data in real time. Comprehensive data observability goes beyond monitoring, by combining it with the ability to manage your data to ensure its accuracy, consistency, and reliability.
Examples of data observability include:
- Lineage Tracking: Tracing data from its source to its final form helps you understand how data is transformed, where you use it, and how various problems are introduced.
- Health Metrics: These measures of quality and reliability are where you implement your data quality framework. Regular monitoring of these metrics can help identify potential issues before they become significant problems.
- Anomaly Detection: With effective data observability, you can proactively identify anomalies and unusual patterns in data. Here again, you put your data quality framework into action with real-time monitoring.
- Metadata Management: Monitoring metadata (data about data) to ensure its accuracy and consistency helps maintain overall data quality and avoid issues such as incompleteness, duplication, and staleness.
- Reporting: The ability to create regular reports covering data health, storage, and collection provides you with a long view of how your data governance efforts are faring.
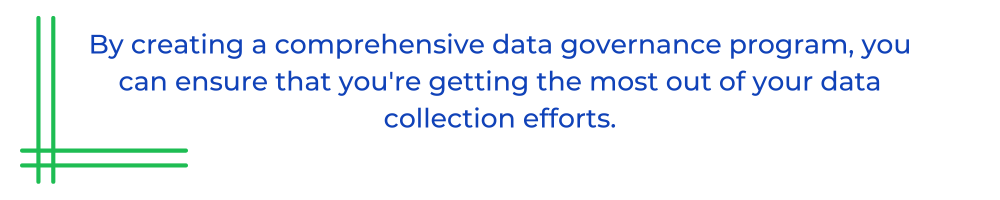
Maintain Data Quality with Acceldata
In this post, we discussed five of the most common data quality issues. Incomplete, duplicated, expired, irrelevant, and inaccurate data will lower the accuracy of your data analysis and can lead you to miss opportunities or make inaccurate decisions.
But you can avoid these problems. By creating a comprehensive data governance program and using the right platform to put it into effect, you can not only prevent data quality issues but also ensure that you're getting the most out of your data collection efforts.
Acceldata is the all-in-one data observability platform for these enterprises. It integrates with a wide range of data technologies, giving you a comprehensive view of your data landscape, as well as the tools you need to observe and manage your data. Contact us today for a demonstration of how we can help.