In today's digital age, data has become the lifeblood of organizations, fueling decision-making processes, powering analytics, and driving innovation. However, the value of data is directly proportional to its quality. Poor-quality data can lead to inaccurate insights, flawed decision-making, and compromised business outcomes. This is where data quality frameworks come into play, offering a systematic approach to ensure that data is accurate, reliable, and fit for purpose.
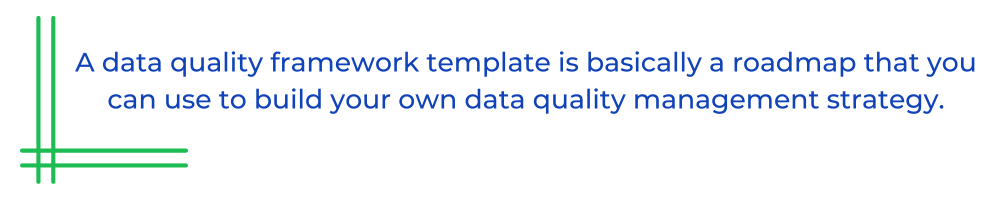
Understanding Data Quality Frameworks
Data quality frameworks provide a structured methodology for assessing, monitoring, and improving the quality of data within organizations. These frameworks encompass a set of principles, standards, processes, and tools aimed at addressing various aspects of data quality, including accuracy, completeness, consistency, timeliness, and reliability.
With a data quality framework, your business can define its data quality goals and standards as well as the activities you are going to take to meet those goals. A data quality framework template is basically a roadmap that you can use to build your own data quality management strategy.
Data quality management is too important to just leave up to chance. If your data quality is negatively impacted, it could have massive consequences on not only the systems that rely on that data but also on the decisions you make in your business.
That’s why it makes sense to develop a practical data quality framework for your organization’s pipeline.
Key Components of Data Quality Frameworks

There are various data quality frameworks, but they typically share common elements:
Data Profiling and Assessment
The first step in any data quality framework involves profiling the data to understand its characteristics, structure, and integrity. This includes identifying missing values, outliers, duplicates, and inconsistencies. Data profiling tools automate this process, providing insights into the quality of the data.
Data Quality Dimensions
Data quality is multidimensional, encompassing aspects such as accuracy, completeness, consistency, timeliness, and relevance. A robust data quality framework defines specific metrics and thresholds for each dimension, allowing organizations to measure and evaluate the quality of their data effectively.
Data Quality Rules and Standards
Establishing data quality rules and standards is crucial for maintaining consistency and integrity across datasets. These rules define acceptable data values, formats, and relationships, helping to enforce data quality standards throughout the organization.
Data Cleansing and Enrichment
Once data quality issues are identified, organizations must take corrective actions to cleanse and enrich the data. This may involve removing duplicates, standardizing formats, correcting errors, and augmenting missing information. Data cleansing tools automate this process, improving the accuracy and reliability of the data.
Data Governance and Stewardship
Data governance refers to the policies, processes, and controls for managing data assets effectively. A robust data governance framework establishes clear roles and responsibilities for data stewardship, ensuring accountability and compliance with regulatory requirements.
Continuous Monitoring and Improvement
Data quality is not a one-time effort but an ongoing process. Continuous monitoring allows organizations to detect deviations from established quality standards and take proactive measures to address them. By continuously improving data quality, organizations can enhance decision-making and drive better business outcomes.
Benefits of Data Quality Frameworks
Implementing a data quality framework offers numerous benefits to organizations:
- Improved Decision-Making: Accurate and reliable data insights enable informed decision-making processes.
- Regulatory Compliance: Ensures adherence to industry regulations and standards by maintaining data accuracy and completeness.
- Operational Efficiency: Reduces time and effort spent on data troubleshooting and manual interventions, enhancing overall operational efficiency.
- Enhanced Customer Experience: Quality data enables personalized and relevant customer experiences, leading to increased satisfaction and loyalty.
Challenges of Data Quality Frameworks
Here are some of the challenges of implementing data quality frameworks:
- Data Silos: Fragmented data across systems and departments pose challenges to maintaining consistent data quality.
- Governance Issues: Lack of clear data governance structures and processes hinders effective data quality management.
- Technological Complexity: Rapidly evolving technology requires continuous adaptation of data quality tools and processes.
- Cultural Resistance: Overcoming resistance to change and fostering a data-driven culture is essential for successful implementation.

With adequate data quality, enterprises can drive outsized financial performance. Data is a crucial asset for incredible profitability and growth. In order to ensure the availability of high-quality and error-free data, a data observability platform is a must.
Implementation Strategies
Implementing a data quality framework requires careful planning, execution, and ongoing monitoring. Some key strategies for successful implementation include:
Assessing Current State
Conducting a comprehensive assessment of current data quality practices, capabilities, and challenges is essential for identifying gaps and opportunities for improvement.
Setting Clear Objectives
Defining clear objectives, goals, and success criteria for data quality initiatives helps align efforts with business priorities and ensures measurable outcomes.
Engaging Stakeholders
Engaging stakeholders from across the organization, including business users, IT teams, data stewards, and executives, fosters collaboration, buy-in, and support for data quality initiatives.
Investing in Tools and Technology
Leveraging data quality tools and technology solutions can streamline data profiling, validation, cleansing, and monitoring processes, enhancing efficiency and effectiveness.
Establishing Governance Structures
Establishing governance structures, processes, and policies for data quality management helps ensure consistency, accountability, and sustainability of data quality initiatives.
Providing Training and Education
Providing training and education to employees on data quality concepts, tools, and best practices enhances awareness, competency, and adoption of data quality practices.
Iterative Improvement
Adopting an iterative approach to data quality improvement allows organizations to continuously assess, prioritize, and address data quality issues based on evolving business needs and priorities.
Common Data Quality Frameworks
Several established frameworks and standards provide guidance and best practices for managing data quality effectively. Some of the most commonly used frameworks include:
TDQM (Total Data Quality Management)
It is a comprehensive approach that encompasses all aspects of data quality management, from data collection to usage. It emphasizes the importance of integrating data quality into organizational processes and systems and involves stakeholders at all levels in data quality initiatives.
DAMA DMBOK (Data Management Body of Knowledge)
It provides a framework for data management practices, including data quality management. It defines key concepts, principles, and activities related to data quality, offering guidelines for implementing data quality initiatives within organizations.
ISO 8000
This one is an international standard for data quality management, providing guidelines and best practices for ensuring data quality. It covers various aspects of data quality, including data accuracy, completeness, consistency, and integrity, and serves as a reference for organizations seeking to standardize their data quality processes.
Six Sigma
It is a quality management methodology focused on minimizing defects and variations in processes, which can be applied to data quality improvement. It employs statistical tools and techniques to identify and eliminate sources of data errors, ensuring data meets predefined quality standards.
Data Governance Frameworks (e.g., COBIT, ITIL)
These frameworks include data quality as one of the key components of data governance, emphasizing its importance in overall data management practices. These frameworks provide structures, processes, and mechanisms for managing data quality across the enterprise.
Optimizing Data Quality: Implementing Data Quality Tools within a Framework
.webp)
Ensuring data integrity is paramount in today's data-driven landscape. Leveraging advanced data quality tools within a comprehensive framework is essential for organizations to maintain high standards. These tools, ranging from data profiling and cleansing to data monitoring and validation, empower businesses to identify and rectify anomalies, inconsistencies, and inaccuracies in their data.
However, effective implementation requires more than just deploying tools—it demands a structured framework encompassing data quality metrics, data governance, and continuous improvement strategies. By integrating these elements seamlessly, businesses can streamline processes, enhance decision-making, and cultivate a culture of data excellence, ultimately driving organizational success.
There are many data quality tools out there. One of the best data quality tools examples is Acceldata. The fact is, your data pipeline is a massively complex series of various systems and tools that carry your data from one place to another.

At any point along this journey, your data quality could be compromised. In order to truly ensure that your data quality remains high, you need an observability solution that monitors your data at each of the key points in your data pipeline. That is exactly what Acceldata is designed to do.One of the great benefits of this kind of monitoring is that you can eliminate issues that impact reliability. This means that you can remove downtime from the equation so that you always have access to your data. These data quality tools can help enterprises to attain the maximum benefits of data governance.
Sometimes, you may hear about or come across open-source data quality tools. These data quality tools have pros and cons that you should be aware of. Open-source tools can sometimes have difficult-to-use interfaces and functionality. This can cost you in terms of additional time and money spent on training.
When building your data quality deck, it’s important to explain how to keep high ratings for all of these metrics. The importance of data quality management is clear. By keeping high levels of data quality, you can ensure that you are always making accurate, insight-driven decisions.
Unifying Data Observability and Quality Frameworks: Elevating Data Integrity
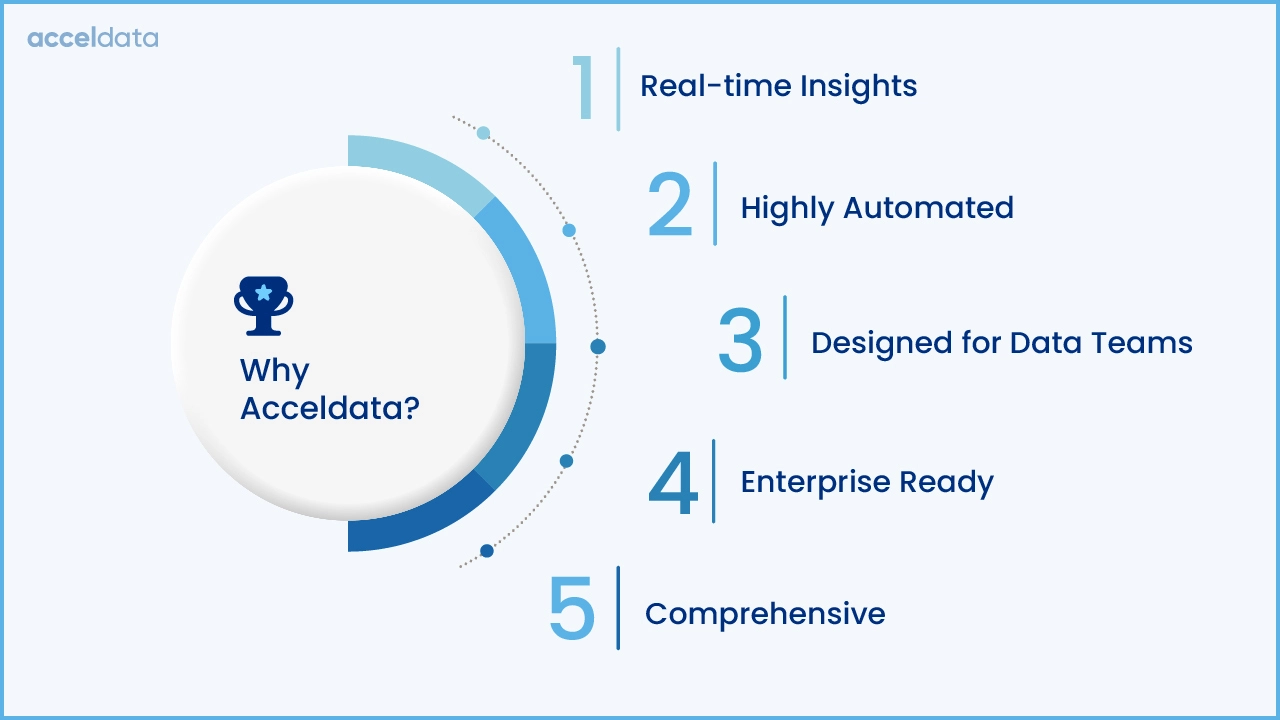
Acceldata offers a comprehensive data observability platform that complements data quality frameworks by providing real-time visibility into data pipelines, workflows, and processes. By monitoring data quality metrics, detecting anomalies, and proactively addressing issues,
Acceldata helps organizations ensure the reliability, accuracy, and integrity of their data. With Acceldata, organizations can streamline data quality management, enhance operational efficiency, and unlock the full potential of their data assets.