How Data Observability Ensures Successful AI & ML Data Products
Artificial intelligence (AI) and machine learning (ML) have been in use by enterprises for decades with many enterprises deploying robust AI and ML applications. There are many processes across a variety of industries, some of which you may encounter as a consumer, that use AI and ML models to perform scoring, make predictions, generate strategic plans, and more.
The new kid on the block is Generative AI which takes AI and ML to an entirely new level of utility. Generative AI offers much greater ease of building AI into applications and creates a more conversational approach. It’s rapidly gaining traction because it allows:
- AI to be embedded in a greater variety of applications
- AI-enabled applications to be deployed more quickly and easily
- Small and medium-sized organizations, with fewer data science skill sets to use AI
Data is the key to allowing AI-enabled applications to work effectively, be trusted, and proliferate. The data fed to AI and ML models must be of exceptional quality in order for them to train and develop those models effectively. This means that data teams have to ensure that the data in their environment is accurate, complete, consistent, relevant, and valid. And once a model is deployed, the data it is fed must also be timely and fresh.
While AI provides endless opportunities, data leaders recognize that nothing can happen unless the data they’re using is reliable. Poor quality data will render AI efforts useless – or worse, it can damage an organization's outcomes. This is where data observability enters the picture.
Data observability helps ensure the highest degree of data reliability on data fed to AI-enabled applications (and across ALL your data) to ensure those applications are reliable, dependable, and produce trusted output. And the Acceldata Data Observability Platform gives you the ability to easily scale your data reliability so you get coverage and consistency across the many different AI-enabled applications you deploy as well as all your other analytics applications.
AI/ML Use Cases
AI and ML are used in a wide range of applications across various industries. Here are some of the most common use cases:
- Natural Language Processing (NLP): NLP techniques enable machines to understand and interpret human language. Common uses include chatbots, virtual assistants, sentiment analysis, language translation, text summarization, and information retrieval.
- Recommendation Systems: AI-powered recommendation systems are widely used in e-commerce, streaming platforms, financial services, and social media. These systems analyze user behavior and preferences to provide personalized recommendations for products, movies, music, articles, and more.
- Predictive Analytics: Machine learning models can analyze historical data to make predictions and forecasts. This is applied in areas such as sales forecasting, demand prediction, fraud detection, customer churn prediction, predictive maintenance, and risk assessment.
- Healthcare and Medicine: AI is transforming healthcare by assisting in diagnosis, drug discovery, personalized medicine, and patient monitoring. Machine learning models can analyze medical images, electronic health records, genomics data, and wearable device data to improve patient care and outcomes.
- Financial Services: AI and machine learning are extensively used in the financial sector for fraud detection, algorithmic trading, credit scoring, risk assessment, customer segmentation, and personalized financial recommendations.
- Industrial Automation: Machine learning algorithms are used for optimizing processes, predictive maintenance, quality control, anomaly detection, and supply chain optimization in industries such as manufacturing, energy, and logistics.
These are just a few examples of the many use cases for AI and ML. We’re really just scratching the surface as we get into how and where it is applied. The field is rapidly evolving, and new applications continue to emerge across diverse industries. While that happens, data teams are finding new uses for how AI will impact their data strategies.
Navigating AI/ML Adoption in Your Data Environment
Organizations face several challenges when adopting and implementing AI and machine learning technologies, including:
- Data Quality and Reliability: AI and ML algorithms require large amounts of high-quality data to train and operate effectively. However, organizations may face challenges in ensuring data quality, timeliness, and consistency.
- Workforce: AI and ML require specialized skills and expertise, especially for data engineering tasks. Acquiring and keeping this talent is critical.
- Regulatory Concerns: Organizations must navigate complex industry and governmental regulatory frameworks to ensure compliance with changing governance requirements.
- Data Integration: Integrating AI and ML into existing infrastructure and data can be complex. Creating and maintaining complex data pipelines is essential to feed AI and ML applications.
- Scalability: Deploying AI and ML solutions at scale is resource-intensive, both in terms of computational power and infrastructure. Organizations may need to invest in powerful data resources to handle large-scale AI workloads.
- Explainability: AI and ML models often operate as black boxes, making it challenging to interpret and understand the reasoning behind their predictions or decisions. Explainability is crucial, especially in regulated domains such as finance or healthcare, where transparency and accountability are essential.
- Security and Privacy: AI and machine learning systems consume a great deal of data and for personalization use cases, there is private data involved. Protecting and governing sensitive data, using data properly, and implementing robust security is essential.
Addressing these challenges requires structured processes, collaboration, strong leadership support, and a focus on building robust governance frameworks.
Data Challenges for Implementing AI/ML
There are several data reliability challenges associated with AI and machine learning. Here are some key areas of concern:
- Data Quality: The models trained by AI and ML require high-quality data. If the training data is incomplete, inconsistent, biased, or contains errors, it can negatively impact the reliability and performance of the models. Ensuring data quality is essential for accurate and trustworthy results.
- Data Bias: Bias in data can lead to biased or unfair outcomes. If the training data reflects historical biases or contains discriminatory patterns, the AI models can perpetuate those biases, resulting in unfair decisions or predictions. Addressing data bias requires careful data collection, preprocessing, and evaluation to mitigate any potential biases.
- Data Quantity and Representation: Sufficient and representative data is crucial for building reliable AI models. In some domains or for rare events, obtaining large and diverse datasets may be challenging. Limited or unrepresentative data can lead to overfitting or underperformance, reducing the reliability of the models.
- Data Drift: Over time, the data distribution that the models were trained on may change, resulting in a phenomenon known as data drift. Environmental or contextual changes can make the trained models less accurate and reliable. Continuous monitoring and adaptation strategies are necessary to detect and mitigate the effects of data drift.
- Data Labeling and Annotation: Supervised machine learning often relies on labeled data, which requires human annotation. The process of labeling data can introduce human errors, inconsistencies, and subjectivity, impacting the reliability of the labeled datasets. Ensuring high-quality labeling and annotation processes is crucial to maintain reliability.
- Data Pipeline Reliability: ML data pipelines that integrate and transform data from various sources, formats, or systems can be challenging. Broken data pipelines can create Incompatibilities, data inconsistencies, or missing data that can affect the reliability of AI models.
That’s a significant checklist, and data teams need all of these things to be addressed continuously. In order to effectively embed these into the discipline of AI and ML requires a comprehensive data observability platform and a rigorous program that ensures data reliability by monitoring and rapidly addressing incidents when they occur.
How Data Observability from Acceldata Ensures Accurate AI
Data observability gives enterprise data teams a single, unified platform to build and manage data products, including AI and ML data products. Data observability helps solve common data pains, including:
- Monitors for, identifies, and delivers alerts about data quality issues and data outages by monitoring data reliability across your data supply chain
- Improves data and analytics platform scaling and eliminates performance issues by identifying operational bottlenecks
- Eliminates cost and resource overruns by providing operational visibility, guardrails, and proactive alerts
The Acceldata Data Observability platform synthesizes signals from multiple layers of the data stack and delivers comprehensive, actionable information so data teams can move fast. It is the only multi-layered solution that provides insights into compute, pipelines, reliability, users, and spend for the data stack.
Acceldata helps data leaders and practitioners solve complex problems involved in building and operating data products, including ones for AI and ML. The platform gives data practitioners and site reliability engineers (SREs) quick insights they can apply to improve data quality, reliability, performance, and efficiency. Data leaders can align their business and data strategies, improve resource efficiency, and increase worker productivity to meet business requirements at a much lower cost.
How Does Acceldata Help with AI and ML?
The Acceldata Data Observability platform answers many of the data challenges that face AI and ML data products. Let’s take a closer look at those:
Data Quality
With a comprehensive approach to data quality testing, monitoring, and alerting, Acceldata ensures that organizations can deliver data of the highest quality to AI and ML models and data products. High-quality data ensures the models make far more accurate decisions and predictions, deliver trust in the model output, and make the data products more effective.
A unique capability of Acceldata is the ability to create User-Defined Functions (UDFs). Data scientists or engineers can take modeling scoring functions and put them into a custom Acceldata data quality policy. This policy can be run each time a data pipeline is executed to give a preview of what the scoring output would look like and check it for accuracy, drift, or other data quality attributes. This becomes a “pre-check” on the model output to prevent potentially bad results from moving downstream into applications.
Acceldata allows teams to easily scale up (add more testing to their data assets) and scale out (add testing coverage to more data assets) through enterprise-level testing performance, templatization, and bulk policy application. Templates and bulk policy application also allows organizations to have greater consistency in their data quality across all their assets.
One Acceldata customer was able to rapidly expand their data quality coverage from five topics to 13, and reduce the processing time on their 500 million rows of data from 15 days for the five topics to less than four hours on all 13 topics.
Data Pipelines
Proper data pipeline execution is critical to developing and operating AI and ML data products. Brittle data pipelines that break can not only slow the flow of fresh data in the data products but can also cause entire parts of the data to be missing causing inaccurate decisions and predictions.
Acceldata monitors your data pipelines, provides detailed execution and performance information, detects anomalies in the execution, and provides alerts when problems occur. Data teams can quickly identify when problems occur, identify where the problem occurred, and resolve the issue. Acceldata also ensures data pipelines deliver timely, fresh data to keep AI and ML data products up to date.
Acceldata also helps data teams shift-left their data reliability to perform quality checks early in pipelines so poor quality or missing data does not impact downstream data products. It facilitates putting circuit breakers in data pipelines to stop the flow of data and allows bad data to be quarantined for troubleshooting by data teams.

Data Drift
Drift is one of the biggest enemies of AI and ML data products. Data drift refers to the phenomenon where the underlying patterns, relationships, and statistical characteristics of the data change over time in the operational environment. This can happen because of undetected upstream data issues or where the data the model encounters in the real world may deviate from the data it was trained on. Either case can cause errors or inaccuracies in the AI and ML data product.
Acceldata offers automated data drift monitoring and detection. When datasets are found to have enough variances, data and data sciences teams are alerted to the issue and provided detailed information about the problem to resolve it. Data drift detection can be applied to data assets but is essentially important in the data going into a model and the output data from a model.
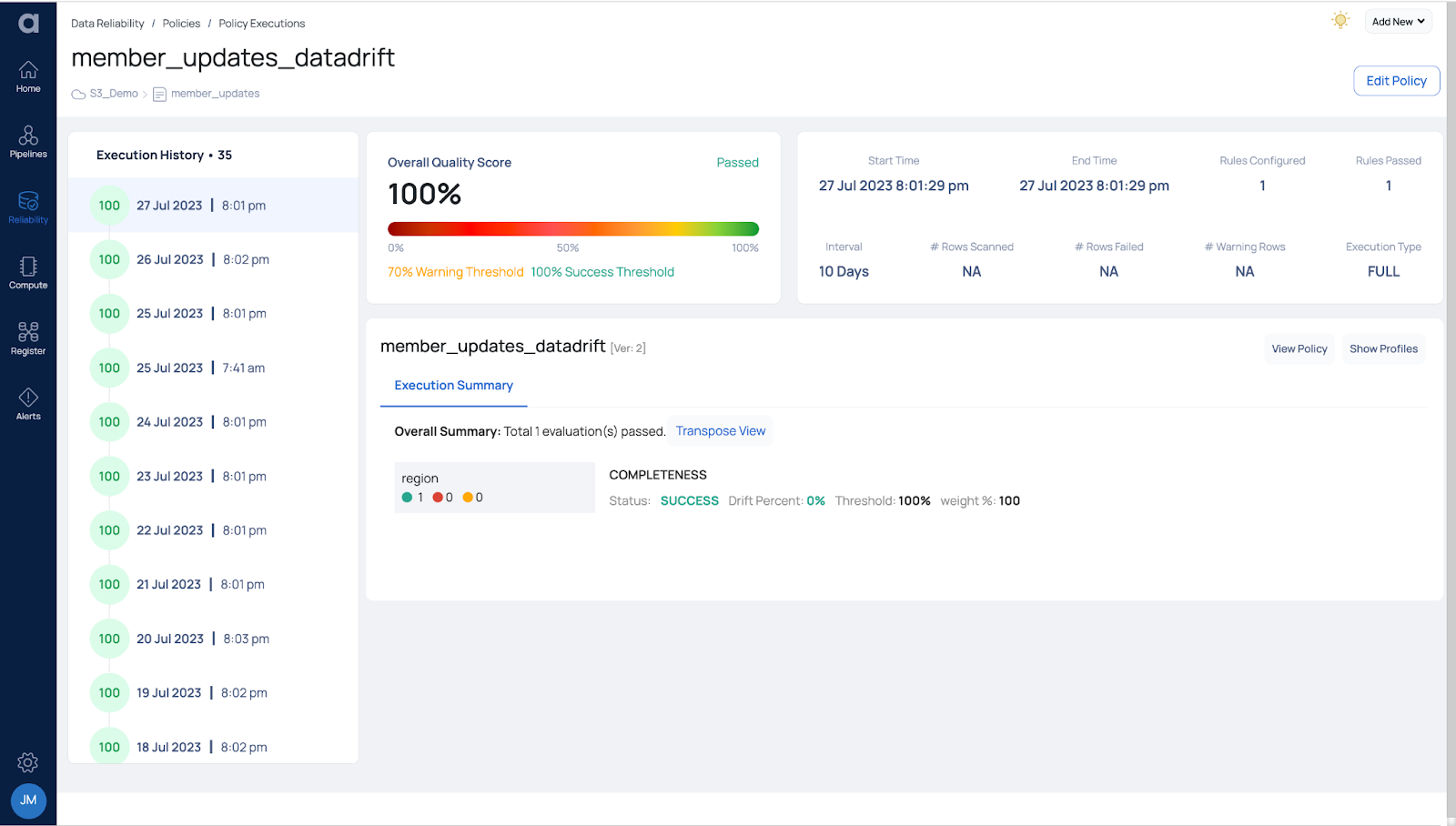
Schema Drift
Schema drift is when a schema is changed in an asset in a data lineage that impacts upstream and downstream assets. Schema drift can cause data pipeline processing to break or create data quality problems due to missing fields or values, which can make AI and ML models produce inaccurate results or not process properly.
Acceldata provides automated schema drift monitoring and detection. When schema drift occurs, data teams are immediately alerted and can fix the issues before data pipelines break or quality issues occur.
Data Reconciliation
The process of data reconciliation compares and aligns data from different sources or systems to ensure consistency, accuracy, and integrity. It involves identifying and resolving discrepancies or inconsistencies between datasets to establish a unified and accurate representation of the data. Data that is not reconciled can cause inconsistencies in the data creating inaccurate AI and ML model decisions and predictions.
Acceldata provides easy data reconciliation policies that are easily applied in a couple of clicks without writing any code. Data reconciliation policies are automated to monitor when problems occur and have alerts to notify data teams where to reconcile the data.
Resource Optimization
AI and ML data products can require and consume large amounts of compute and data platform resources. Platform engineers need to understand, plan, and optimize the available resources to ensure that AI and ML data products continue to operate effectively.
Acceldata’s Operational Intelligence capabilities apply to all phases of the AI and ML data product development process. It optimizes solution design by analyzing designs and workload impact across your entire data stack. Deployment is simplified by tuning for scale with bottleneck analysis, configuration recommendations, and a simulator. Post-deployment, real-time insights, and alerts monitor ever-changing workloads and provide recommendations to tweak configurations on demand.
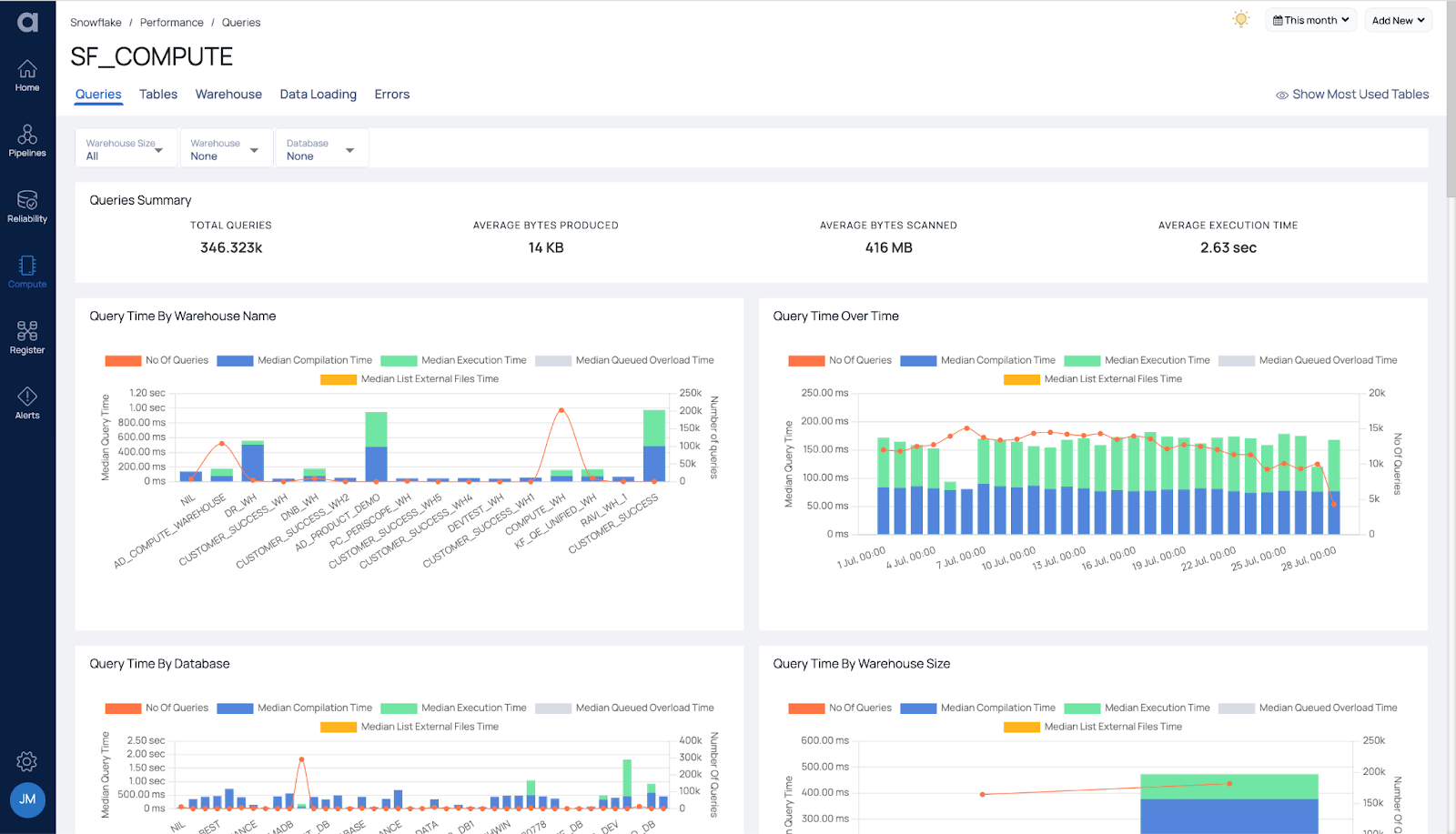
Cost Management
A critical element to determining the ROI of AI and ML data products is to understand the costs consumed within the data platforms. FinOps teams need detailed data on cost consumption so they can allocate those to specific AI and ML solutions as a piece of the ROI calculation.
Acceldata provides the breadth and depth of insights about utilization and associated costs for your cloud data platform. It supplies cost insights from multiple angles, which allows data teams to explore and track costs across multiple aspects. Acceldata also provides cost forecasting, guardrails, and recommendations for effective planning, the elimination of cost overruns, and the optimization of platform resources.
Alerts & Incident Management
Acceldata is designed with a strong operational focus to help data teams continuously monitor, remedy issues, and optimize their data assets, data pipelines, and data infrastructure to ensure the highest degree of data health, manage and control costs, and deliver highly tuned services to business teams.
The platform supports an incident management and alerting framework which spans the core pillars of data observability provided by the platform - these include real-time spend and performance monitoring of data platforms, data reliability (quality, reconciliation, data drift, schema drift) monitoring of data assets, and real-time monitoring of data pipelines.
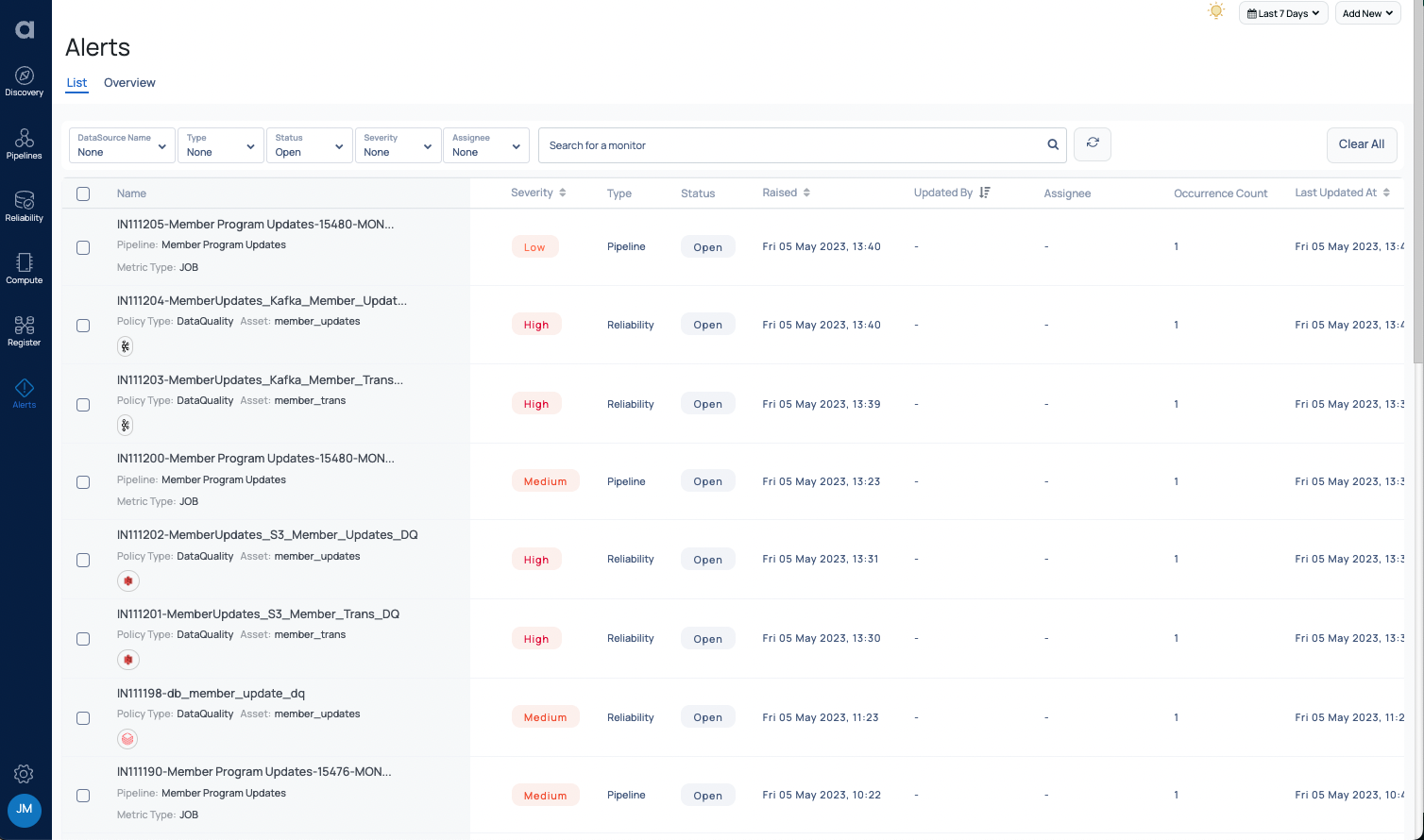
Besides tracking all four areas of data health, Acceldata’s multi-layer data system manages a rich repository of granular data from each area. All this data is correlated to provide 360-degree views into what is happening with your data and give data teams the ability to drill down into the data to investigate issues and find ways to improve.
Acceldata also provides detailed context through charts and analytics. These capabilities help monitor pipelines, data reliability, spend, and data infrastructure performance. The platform also includes out-of-the-box and configurable monitors so team members can be alerted to issues when they occur as well as regular updates on job execution. Issues are also managed and tracked within the system.
Using Data Observability to Drive AI Initiatives
Data observability is essential to successful AI and ML data products. Data observability platforms ensure high data quality, properly executed data pipelines, and effective resource allocation so AI and ML models work effectively, to specification, and within ethical and regulatory guidelines to produce accurate decisions and predictions for maximum impact.
Acceldata provides the industry’s most comprehensive and enterprise-grade data observability platform. With robust data reliability, spend intelligence, and operational intelligence Acceldata ensures your AI and ML data products are fed high-quality data, data pipelines deliver timely data, data does not drift and is properly reconciled, and resources are properly allocated to meet operational and cost requirements.
Interested in seeing Acceldata in action? Please schedule a personalized demonstration.