Just about every modern data environment relies on Snowflake as a way to make more sense of its data. Snowflake is a cloud-based data warehouse and analytics platform that’s designed to provide an easy-to-use, elastic, secure and cost-effective solution for managing large amounts of structured and unstructured data. Snowflake provides a wide range of features, including scalability, high availability, performance optimization, and more.
Throughout the entire data process – from ingestion to consumption – data pipelines are moving data from disparate sources in an attempt to deliver actionable insights. When that data is accurate and timely, those insights help the enterprise gain a competitive advantage. In other words, they deliver on the promise of the data-driven organization. But there’s a lot that has to happen for enterprises to optimize their data performance and that starts with ensuring that data is reliable. Organizations that seek to achieve data reliability generate continuous visibility into the efficacy of their data and data pipelines and can detect and remediate issues early in the data journey.
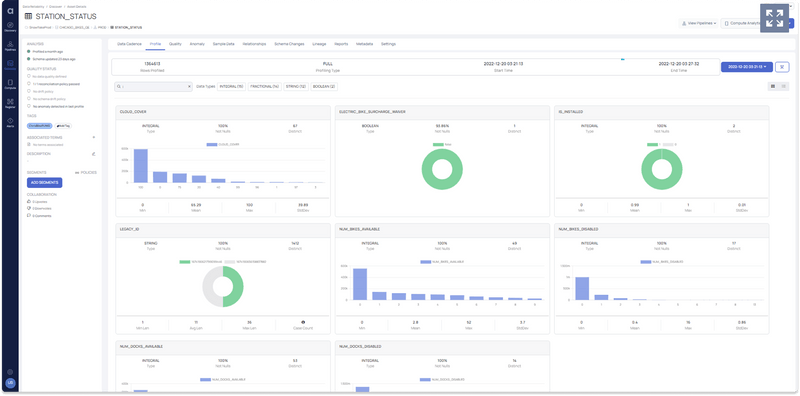
There’s no question that Snowflake environments can be complex; relying on just data quality is insufficient. A data reliability-driven solution like Acceldata’s Data Observability platform, helps data teams to isolate data issues in all areas of Snowflake operations. To understand where this happens, we need to first take a closer look at how Snowflake is structured.
Snowflake Data Quality Framework
Data quality frameworks are essential for organizations to ensure that their data is accurate, reliable, and secure. A data quality framework provides a set of guidelines and processes to help organizations manage their data in an efficient and effective manner. It helps organizations identify potential issues with their data, develop strategies to address those issues, and monitor the results of those strategies over time.
Snowflake provides guidance for a data quality framework, and combined with an approach to data reliability, like that provided by the Acceldata Data Observability platform, data teams maximize the data in their Snowflake environments by ensuring timely, fresh, and quality data.

If you know the Python scripting language, you can also write your own Python data quality framework with all the rules and specifications you need to achieve your goals. Snowflake provides the “Snowflake Connector for Python,” which enables you to create Python applications that can connect to Snowflake to perform operations.
Because of the importance of data quality and data governance, Snowflake has created the Snowflake Data Governance Accelerated program. This program is designed for Snowflake data governance partners who have developed solutions that can integrate with Snowflake and enhance its already robust governance capabilities.
Snowflake Data Profiler
Data profiling is another important step in ensuring that your data is accurate and reliable. It involves analyzing the structure of a dataset by looking at things like column types, missing values or outliers, etc. With Snowflake, you have access to open-source libraries such as Pandas-Profiling or the data-profiling Github library, which allow you to quickly profile your datasets without having to write custom code from scratch each time. You can also use the Snowflake ‘Profile Table’ feature, which gives an overview of all columns within a table, including their type, size, null value counts, etc. This helps identify any potential issues with the dataset before running further analysis on it.
Snowflake Data Governance
Snowflake Data Governance is a cloud-based platform that provides organizations with tools for managing their data assets in a secure and compliant manner. The platform enables users to define policies for access control, audit trails, encryption, masking, classification labels, and more. Additionally, it provides an intuitive user interface for creating catalogs of data sources and visualizing relationships between them.
Snowflake Data Freshness
One key benefit of using Snowflake’s Data Governance offering is its ability to help keep your datasets fresh by enabling you to monitor changes over time via real-time observability tools. This allows you to quickly identify any discrepancies between different versions of datasets, ensuring accuracy across all reports/documents produced within your organization while also reducing manual effort associated with tasks like manually reconciling differences between two versions of the same dataset every month/quarter, etc. Snowflake data analysis can be a powerful way to help you take control of your big data. With Snowflake data types categorization and Snowflake data visualization, you can achieve even better visibility. However, using Snowflake monitoring or Snowflake data sharing can sometimes be challenging. A data observability solution can help you democratize access to critical insights.
With Acceldata Data Observability solutions, data teams can enhance Snowflake’s capabilities. Learn more here about how Acceldata and Snowflake can help you go farther together.
Photo by Shubham Dhage on Unsplash