The COVID-19 crisis is creating unprecedented need and demand for information sharing.
Decision-makers today require more context than their own organization, databases, standards, and well-trodden communication channels can supply. For example, UK regions with previously disconnected mandates now exchange data with one another on the availability and utilization of critical equipment, such as ventilators, across their respective hospital networks.
Organizations across the board are having to deal with the challenge: how to provide this cross-cutting broad information access.
As corporations and governments modernize their data architecture — to close this gap between information and outcomes — they are presented with numerous choices now — using a combination of data warehouses, data lakes, and their cloud counterparts.
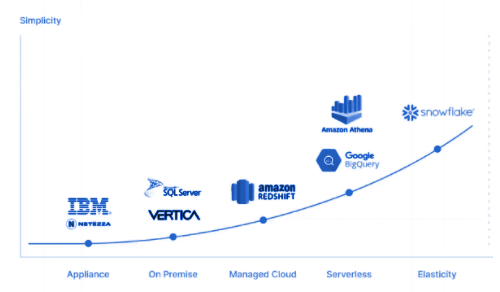
The evolution of data warehouses in the last decade has changed the way enterprises evaluate technology. Earlier, the data warehouse evaluation criteria used to be price/performance metric.
However, with the explosion of unstructured and semi-structured data, a single solution (data lake or warehouse) cannot handle the big gorilla in the room — that is data variety.

In essence, we believe that there is no one size fits all for data warehouses and data lakes.This is a rapidly evolving landscape, cloud data lakes are adding SQL analytics capabilities (e.g. Databricks’ SQL Analytics) and cloud data warehouses are adding more data lake-like features. Data lakes are better suited when an organization has a lot of data variety.

We find that regardless of the route you choose, it’s important to not get into these data pitfalls:
1) Not aligning with your organization’s data transformation journey
If your company only uses one or two key data sources on a regular basis for a select few workflows, then it might not make sense to build a data lake from scratch, both in terms of time and resources.
See the table above to understand which dimensions fit your organization.
Ultimately, this boils down to prioritizing long-term value and scale of data/business intelligence investments over one-off wins that are isolated across the enterprise. It is critical to understand that scale comes from building a healthy data foundation which involves back-end decisions made around how data is managed, as well as front-end decisions around how it is analyzed and visualized. Issues arise almost always from treating the back end and the front end as architectural silos and not getting the fundamentals right from the ground up.
2) Lack of collaboration from the ground up
The problem with many data projects is that the business plays a marginal role in the way data-driven workflows are built.
While business representatives may be present on joint project teams, they rarely have access to a collaborative platform through which they can truly iterate on both the data assets and the key workflows.
When business users are not first-class participants in building a data foundation and the associated workflows, they stop using it. As a result, it is not uncommon to see more IT staff dedicated to building an end-to-end solution than to using it regularly.
In the big data universe, there is a lot of chatter about “data silos” but an equally pervasive challenge is the hidden silos of tribal knowledge. In fact, many companies still operate within a spreadsheet culture in which users extract data from internal systems, load it to spreadsheets and perform their own calculations without sharing them company-wide. defining key business metrics transparently in a common data foundation.
The metrics can then be enriched across the enterprise several times as long as the organization retains the ability to track each revision along the way. In this sense, the abilities to write back new data transformations into the data foundation and trace their lineage are critical components of a sustainable data architecture.
3) Insufficient rigor in tackling enterprise-wide data integrity
Data warehouse, data lake, data lake-house: it doesn’t matter if you can’t trust your data.
A key aspect of this is the notion of kaizen or continuous improvement — from the perspective of data integrity. Can business users flag data quality issues on the analysis platforms as soon as they encounter them? Can data engineers address the issues flagged by users in near real time? Are users and engineers able to dive into the lineage of the data to understand where quality issues originate?
Avoid Data Pitfalls with Data Observability
Your thoughtful investment in the latest and greatest data warehouse doesn’t matter if you can’t trust your data. To address this problem, some of the best data teams are leveraging data observability, an end-to-end approach to monitoring and alerting for issues in your data pipelines.
Previously valid assumptions about economies, businesses, and societies may no longer be valid, decision-makers and operators around the globe seek broad contextual information to prepare for an uncertain future and data analytics are at the forefront of this transformation.