A Fortune 100 company recently saw its customer churn prediction model fail the day before launch. The culprit? A broken ingestion pipeline that went undetected. Not the AI model. Not the strategy. Just brittle, reactive data plumbing.
As Chief Product Officer at Acceldata, I’ve seen how even the most advanced enterprises stumble—not due to lack of ambition, but because of fragile, disconnected infrastructure. Traditional observability tools gave us visibility, but left us drowning in dashboards and triaging alerts manually.
That’s why we’re rearchitecting the system itself. Agentic Data Management (ADM) is not just a product—it’s a paradigm shift. ADM introduces autonomous intelligence into the data layer: agents that detect, reason, and resolve without human intervention. With ADM, your data ecosystem becomes a living, adaptive system—always on, always aligned to business needs.
Why Traditional Data Ops Fall Short—and Where Business Suffers
Today’s data stacks were built for batch processing—not AI. Enterprises struggle with siloed catalogs, reactive observability tools, and manual governance workflows. The result?
- Decision latency
- Data downtime
- Broken trust across business units
ADM changes the model. As I shared on the Data Hurdles podcast, it brings cognition into data operations. Think intelligent agents—not just monitoring, but resolving issues with human-in-the-loop learning before problems snowball into revenue or compliance risks.
"You can't automate intelligence if your pipelines are still manual and brittle."
— Ramon Chen, Chief Product Officer, Acceldata
What ADM Does: Real Autonomy Across Your Data Estate
Coordinate Smarter: Automate Across Fragmented Systems
ADM agents orchestrate workflows across Airflow DAGs, Spark jobs, Snowflake tables, Kafka streams, and more. The result? Eliminated bottlenecks, resilient pipelines, and SLAs that hold.
Example: A global bank uses ADM to detect DAG failures in Airflow and auto-reroute Spark workloads to meet SLA thresholds—without waking up engineers at 2am.
Ensure Quality that Never Sleeps
Traditional tools alert. ADM resolves. Its AI agents enforce lineage, validate pipelines, and protect downstream models.
Example: A leading e-commerce company uses ADM to catch schema drift in Snowflake and isolate anomalies affecting their recommendation engine—ensuring personalization never breaks.
Translate Technical Issues Into Business Impact
ADM’s xLake Reasoning Engine learns from interactions, mapping anomalies to KPIs, and connecting technical issues to revenue risk or operational slowdowns.
Example: A healthcare payer identifies upstream message queue congestion using ADM and quantifies its downstream impact on claim approval timelines—triggering automated fixes.
The Blueprint for Autonomous Data
To realize true autonomy, you need trust, explainability, and adaptability. ADM was built with this in mind:
- Trustworthy Data Agents: Transparent lineage, embedded governance
- Business Notebook: Natural language interface for analysts and ops teams
- Agent Studio: Build custom agents tailored to enterprise logic—no code required
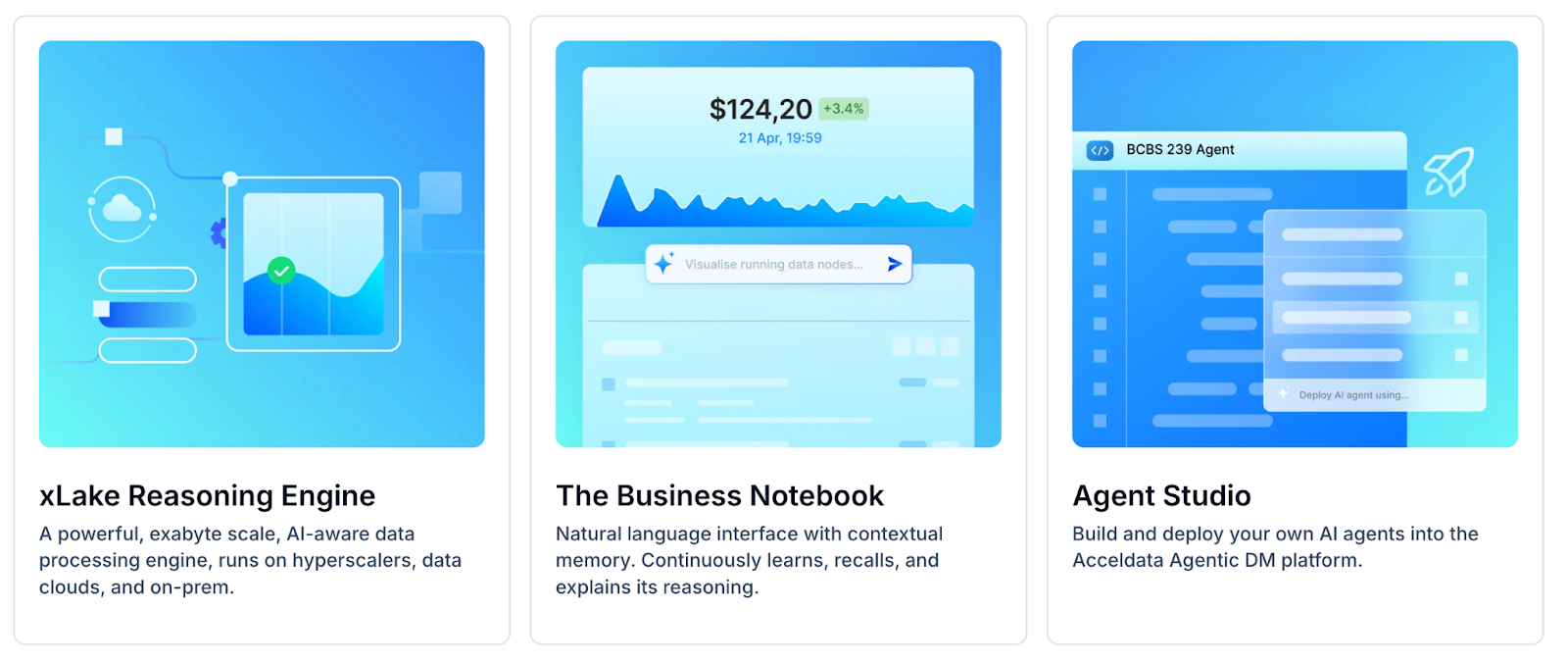
"Think of ADM like an AI co-pilot for your data team—always optimizing, always learning." — Ramon Chen, CPO at Acceldata
Final Thoughts: From Reactive Ops to Strategic Intelligence
Data complexity isn’t destiny. With ADM, we don’t just get visibility—we get velocity. We don’t just monitor data—we act on it.
This is the AI-native foundation that enterprises need. One where your models aren’t second-guessing your pipelines. One where governance, observability, and optimization converge.
ADM is more than a product. It’s a category-defining shift. Let’s make your data unstoppable.