Download the PDF version: 3 Ways to Optimize Your Snowflake Spend
Analyzing How Snowflake Data Management Affects Your Overall Compute Costs
Snowflake is one of the more popular SaaS platforms offering data warehousing capabilities and services. Most service providers either charge a flat fee or base their costs upon the services rendered, and Snowflake is no exception to this rule. As a flexible cloud-computing service provider, Snowflake’s architecture allows a user to control and manage costs by supervising three distinct functions:
- Storage
- Compute
- Cloud Services
Yet, as Snowflake users understand all too well, visibility into the data activity happening within their Snowflake environment can be challenging.
This can present a number of data performance, data quality, and economic issues.
Without the ability to see how data is functioning, and unless there is awareness of the cost impact of operating within Snowflake, data teams run the risk of overspending or underutilizing this critical element of their data stack.
This paper offers insights into how data teams can optimize the performance and cost management of their Snowflake environments with greater visibility.
As we will see, using data observability is now recognized as among the most important factors in this effort, and we will demonstrate how employing data observability within Snowflake environments is helping data teams of all sizes improve the cost and performance of their data investments.
The compute and cloud services stages of Snowflake’s service can be broadly defined as the process of loading data into the virtual warehouse and executing queries. This is billed to the customer in the form of Snowflake credits.
Data storage on the other hand is charged using a flat-rate fee that’s based on expected monthly usage.
Customers can purchase storage and Snowflake credits on-demand or up-front depending on the working capacity.
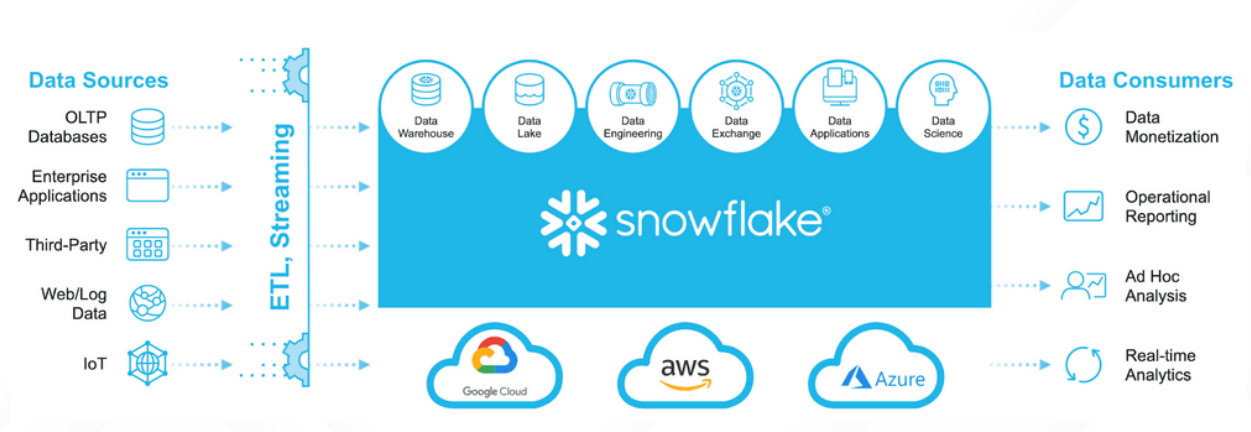
Understanding How Much You Pay for Snowflake
Storage Costs
Calculating storage costs is one of the more straight forward pricing models of the Snowflake platform.
Most customers are billed a standalone fee of around $23/TB of data, which is accrued daily. However, we must also take into account that different customers pay slightly offset rates depending on their contractual agreements and obligations with Snowflake. For example, a customer using Azure in Zurich, Switzerland, would pay a fixed rate of $50.50 per TB/month, while another in Washington would pay $40 per TB/month on demand.
The Cost of Compute
Snowflake costs are calculated either on demand or by pre-purchasing capacity. Paying on-demand means you pay for only the services you consume. So, Snowflake calculates the total cost of the resources you provisioned for a month, and then you pay in arrears.
This is where the Snowflake credit system comes into play. Each credit is pegged at a certain rate, which could range from anywhere between $2.50 - $6.50 per credit. This range purely depends on the region, function-criticality and cloud service provider.
Virtual Warehouse Credit Usage
Simply put, this is the cost incurred when you execute queries, load data or perform data operations on multiple compute clusters. The total cost is factored into the size of a warehouse the query is run upon and the total time taken to run the query.
On the other hand, Snowflake also offers customers data warehouses of different sizes, where you can run queries on increasingly more powerful warehouses for optimum performance.
The downside is that the credits used per hour depend on the size of the warehouse, which is illustrated below.
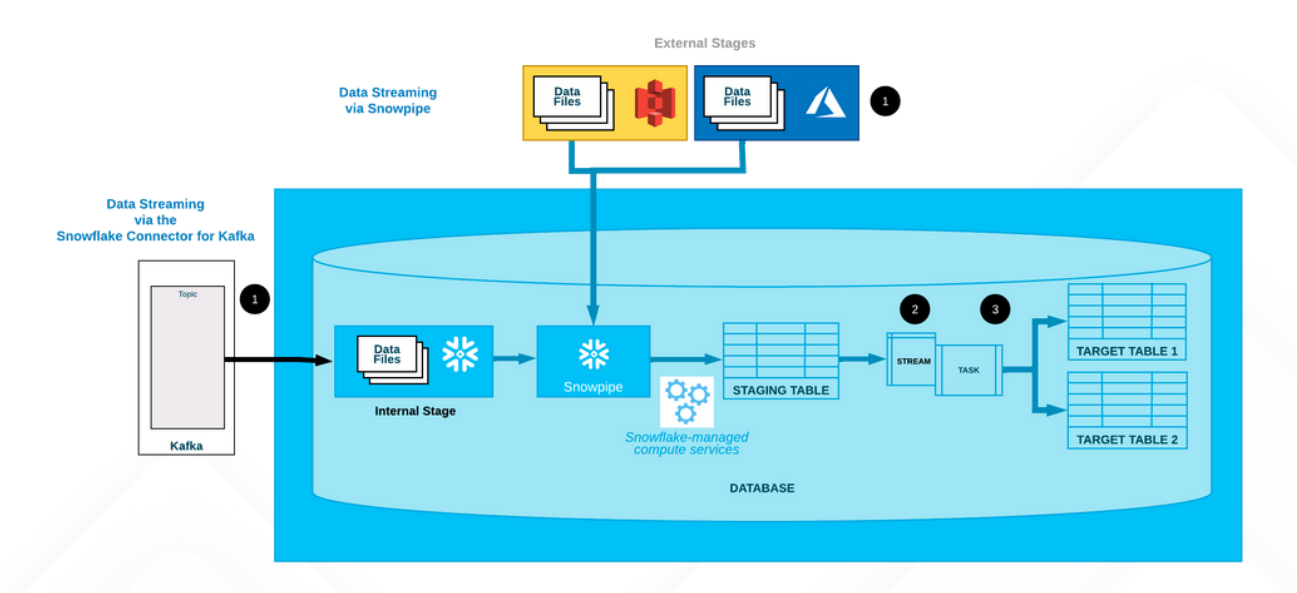
In an attempt to derive the cost of running a single query, we need to take the credit usage/hr cost given above and multiply it by the elapsed time (T) taken when running the query.
This value is then multiplied by the cost of each credit, which is defined in the customer agreement.
Considering the overall expense of running a data environment, this number may seem significantly minuscule. However, as the size and scale of your operations expand, so do the costs exponentially.
Large enterprises and conglomerates can run thousands of queries per day, taking the overall costs to new heights.
Credit Usage = (Credit Usage/Hour)(Elapsed Time [in hours])(Cost Per Credit)
For the above equation, let’s consider a scenario with a query running on an extra large warehouse for 300 seconds. We must remember that since time is taken in hours, T = 300/3600 = 0.083 hours. Also, since each credit cost varies, let’s presume it to be $3. Therefore, credit usage cost = 16 x 0.083 x 3 =$3.98/query.
Note: Even if a single query runs for only five seconds, Snowflake charges a minimum of one minute.

Straightforward Pricing, Unpredictable Outcomes
The entire pricing system that Snowflake employs is quite easy to comprehend you pay for what you use. However, when we talk about data and distributed data systems, the concept of cost may begin to seem skewed.
Today, an enterprise gets data in all forms and shapes from diverse sources. The volume of data flowing into each data warehouse or data lake can fluctuate without any warning.
As an organization grows and adds more layers to its operations, verticals and customer-facing applications, data pipelines are relied on for more activity and become harder to manage.
Businesses can only derive value from data if actionable insights from that data lead to better decision-making.
To ensure the best possible outcomes, the entire data pipeline must be monitored with precision. With every single query being billed to the customer, any breakdowns in data pipelines (or ETL of poor quality/erroneous data) must be addressed and rectified immediately to ensure maximum ROI from Snowflake investments. Whether you pay on demand or pre-purchase capacity, you can prevent wastage by consistently observing and optimizing your data systems using data observability tools.
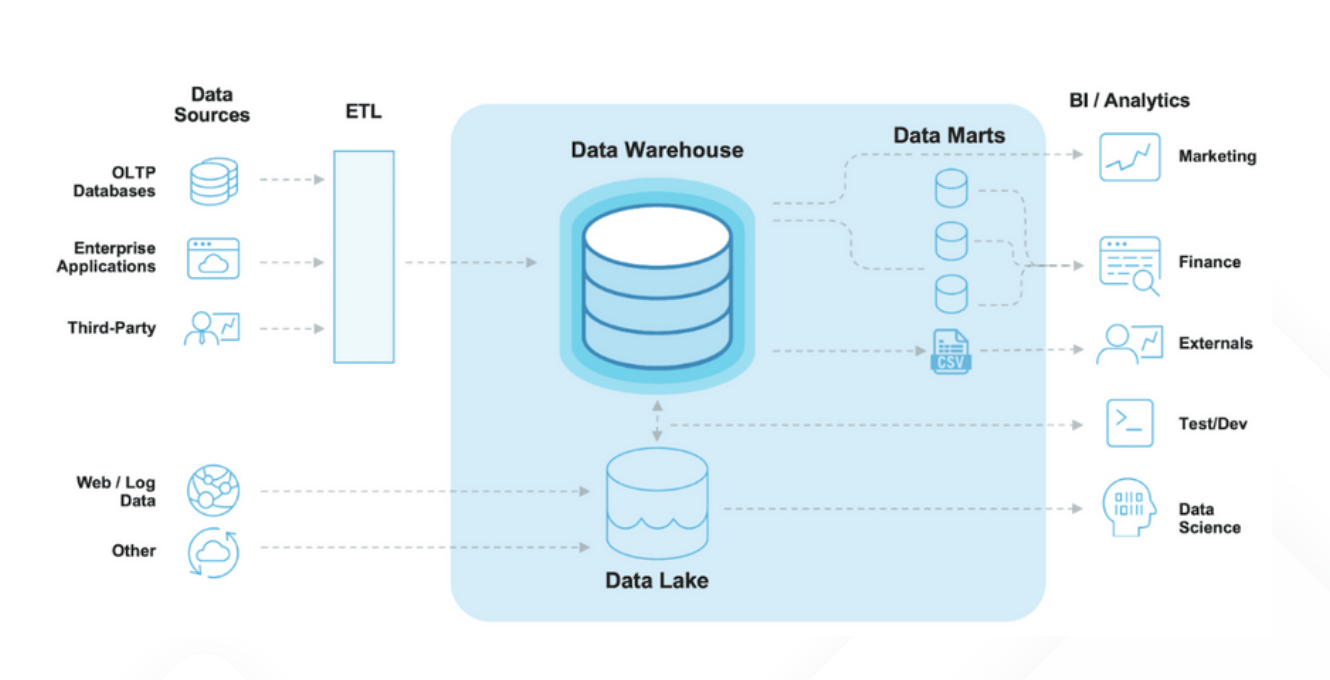
What is Data Observability?
Gartner defines data observability as “the ability of an organization to have a broad visibility of its data landscape and multilayer data dependencies (like data pipelines, data infrastructure, data applications)at all times with an objective to identify, control, prevent, escalate and remediate data outages rapidly within expectable SLAs.” It describes your system’s capacity to find, analyze, and correct data problems in near real time using various technologies and methods. Data observability helps you detect and identify the primary causes of data discrepancies and provides preventative steps to improve the efficiency and reliability of your data systems.
The data that is used to make decisions within an enterprise must, in essence, accurately describe the real-world (and must reflect the continuous activities and influences that impact the enterprise’s environment). To do that effectively, data must exhibit six necessary characteristics. Data observability aims to help organize and surface high-quality data that is:
- Accurate: Is the correct account balance a dollar, or is it a million dollars? Inaccurate data is meaningless, and even worse, can lead to costly, time-consuming errors.
- Complete: Does the data in question describe a fact completely, or is it missing key elements? Without complete data, an organization is unable to see all facets of the issues they encounter.
- Consistent: Is the data being used for a given purpose consistent with the related data points stored elsewhere in the system? There has to be some relationship between and among data elements, and these must be identified and used appropriately.
- Fresh: How old is the data element? Does it still reflect current business realities, or is it stale and no longer representative of the current status? Stale data can lead to bad, erroneous decisions.
- Valid: Does data conform to schema definitions and follow business rules? If data sets cannot communicate because they are invalid, you will only see partial information.
- Unique: Is there a single instance in which this information appears in the database? Multiple instances can lead to version control issues and gaps in data.
In order to ensure these six data requirements, organizations need to establish checkpoints across the entire data pipeline.
This helps eliminate data reliability challenges and provide early warnings if there are data quality issues.
How Data Observability Helps Reduce Snowflake Costs
Monitors Resources for Deviations from Standard Practices and Optimizes Resource Performance
Standard practices help optimize a business’s data resources and ensure its data quality. For instance, enabling auto-suspend for all your virtual warehouses would automatically pause the warehouses when they’re not processing queries. You could also filter out data that should not be processed so that you reduce the amount of work done.
With data observability, businesses can receive alerts whenever they no longer follow set standards or best practices. For example, it can send notifications when you’re processing data when you should not — or not including timeouts for your requests and queries.
Data observability also helps analyze and manage bottlenecks, high data spillage, compilation time, data volume, resource allocation, and other aspects of data quality and management. It helps ensure that your resources are working as intended with low latency.
Improves Resource Efficiency
Data observability helps detect overprovisioning of Snowflake services and performance inefficiencies.
For example, you can have a large warehouse with fewer data. And the larger your warehouse is, the more Snowflake credits you’ll use to process requests — however, the larger your warehouse, the faster the response.
By gaining insight through data observability, you can compare the pros and cons of larger and smaller warehouses and make adjustments based on your business needs.
Data observability also allows businesses to detect spikes and queries that run too long, providing recommendations where needed.
Provides Cost Intelligence
High cloud spend amounts are often due to unused or overused cloud resources. For example, you could accidentally run test code on demand, which does your business no good. Or you could pre-purchase a lot more capacity than you need.
Want to know which resources you should provision? Or how much it will cost? Data observability can help.
It checks how large your data is(volume), how often you update it (freshness), and where and how often you access it (lineage) to offer recommendations on the most efficient way to use Snowflake’s services.
Data observability tools also help businesses forecast their Snowflake costs so they can review, make changes, and plan accordingly.
Acceldata: Data Observability for Your Snowflake Environments
For data to be valuable to a business, it needs to be reliable, accessible, and trustworthy. The good news is you can pay for what you need and optimize your cloud resources to fit your budget and computing processes.
Start leveraging data observability in your data systems and structures to reduce your Snowflake costs.
With Acceldata, you can track your data pipeline performance and quality inside and outside of Snowflake, thus optimizing your Snowflake costs.
Acceldata Data Observability Cloud helps companies follow Snowflake’s best practices, and when they’re in violation, Acceldata sends notifications and recommendations.
Using Acceldata provides the insights that data teams need to develop a contract plan, current and projected spend analysis, department-level tracking, budgeting, and chargeback. Acceldata also helps you avoid the effects that often accompany businesses when they switch to cloud platforms.
Acceldata can help migrate quickly and affordably to Snowflake by providing visibility and insight to raise your data’s efficiency and reliability and reduce cost. If you need help figuring out how to begin, Acceldata offers a simple and effective integration that gets you started quickly.
See Acceldata in Action
- Gain control: Ensure resources are used efficiently and with guardrails to align cost to value
- Build data trust: Deliver high quality data, on time, every time
- Get best practices: Monitor and analyze performance and configuration to get the most from Snowflake.
Get a demo of Acceldata to learn how to maximize the return on your Snowflake investment with insight into performance, quality, cost, and much more.
Download the eBook: 3 Ways to Optimize Your Snowflake Spend